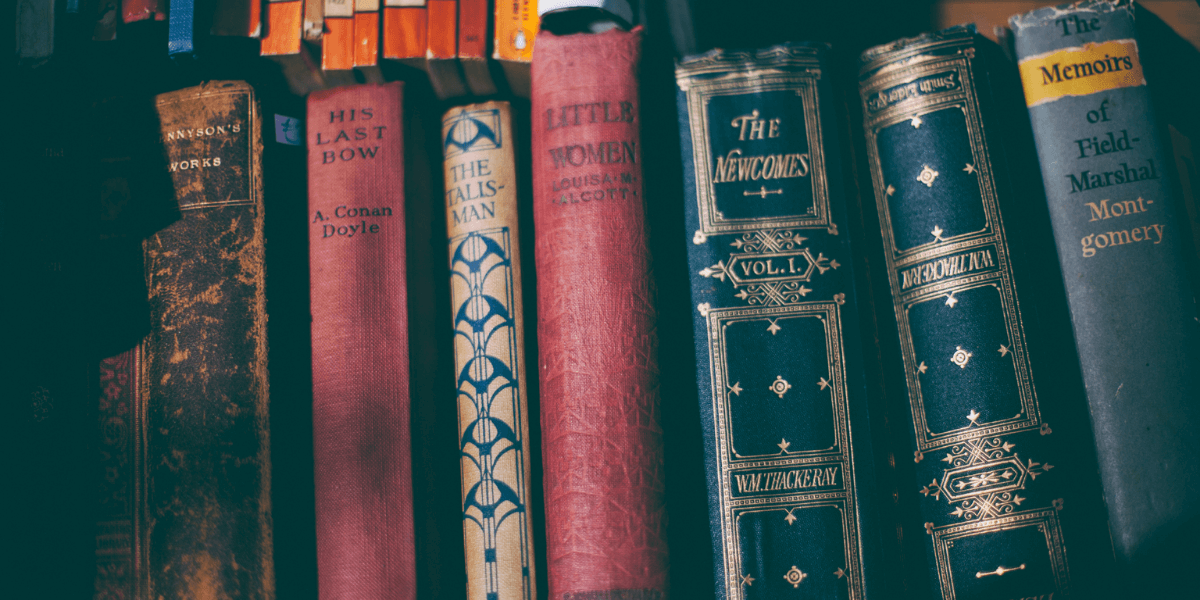
Clem Onojeghuo/Pexels
Characters like Jo March and Dana Franklin have been rare in literature.
Now, researchers at the USC Viterbi School of Engineering have utilized AI technologies to conclude that male characters are four times more prevalent in literature than female characters like Jo and Dana.
Mayank Kejriwal, a research lead at USC’s Information Sciences Institute (ISI), was inspired by current work on implicit gender biases and his own expertise in natural language processing (NLP). While many published studies survey and analyze the qualitative aspects of female representation in literature and the media, Kejriwal’s research particularly made use of his strengths — collecting quantitative data through existing machine learning algorithms.
To produce these findings, Kejriwal and Nagaraj accessed data through the Gutenberg Project corpus which contains English-language 3,000 books, an added attempt to mitigate researcher bias. The genre of books ranged from adventure and science fiction, to mystery and romance, and in varied mediums, including novels, short stories, and poetry.
Akarsh Nagaraj, M.S. ’21, co-author of the study and Machine Learning Engineer at Meta, helped uncover the 4:1 male-female literary imbalance.
“Gender bias is very real, and when we see females four times less in literature, it has a subliminal impact on people consuming the culture,” said Kejriwal, a research assistant professor in the Daniel J Epstein Department of Industrial and Systems Engineering. “We quantitatively revealed in an indirect way in which bias persists in culture.”
Nagaraj noted the importance of how their methods and the study’s findings imparted them with a greater understanding of biases in society and its implications. “Books are a window to the past, and the writing of these authors gives us a glimpse into how people perceive the world, and how it has changed.”
Men everywhere…and main characters
The study outlines several methods for defining female prevalence in literature. They utilized Named Entity Recognition (NER), a prominent NLP method used to extract gender-specific characters. “One of the ways we define this is through looking at how many female pronouns are in a book compared to male pronouns,” said Kejriwal. The other technique is to quantify how many female characters are the main characters in it.
This allowed the research team to determine whether the male characters were central to the story.
The study’s findings also showed that the discrepancy between male and female characters decreases under female authorship. “It clearly showed us that women in those times would represent themselves much more than a male writer would,” said Nagaraj.
The team’s diversified methods to measure and determine female representation in literature did not come without limitations, however, when authors are neither male or female. “When we published the dataset paper, reviewers had this criticism that we were ignoring non-dichotomous genders,” said Kejriwal. “But we agreed with them, in a way. We think it’s completely suppressed, and we won’t be able to find many [transgender individuals or non-dichotomous individuals].”
Challenging dichotomies
Kejriwal acknowledged that AI tools for identifying plural words, such as “they,” which may be referring to a non-dichotomous individual, do not yet exist. Still, the study’s findings build the framework for approaching such social issues and building the technologies that can address these deficits.
The study also provides a blueprint for future work on quantifying the qualitative findings they discovered through the study’s methodologies. Without the inherent bias from human-designed surveys, the NLP technology also enabled them to find adjective associations with gender-specific characters, deepening their understanding of bias and its pervasiveness in society.
“Even with misattributions, the words associated with women were adjectives like ‘weak,’ ‘amiable,’ ‘pretty,’ and sometimes ‘stupid,’” said Nagaraj. “For male characters, the words describing them included ‘leadership,’ ‘power,’ ‘strength’ and ‘politics.’”
While the team didn’t ultimately quantify this facet of their study, this difference in qualitative descriptions between gender-specific characters provides future scope for more comprehensive qualitative investigation on word associations with gender.
“Our study shows us that the real world is complex but there are benefits to all different groups in our society participating in the cultural discourse,” said Kejriwal. “When we do that, there tends to be a more realistic view of society.”
Kejriwal is hopeful that the study will serve to highlight the importance of interdisciplinary research — that is, using AI technology to highlight pressing social issues and inequalities that can be addressed. Stakeholders with specialized backgrounds, including computer scientists, can offer tools to process data and answer questions, and policymakers can use this data to enact change.
Published on April 22nd, 2022
Last updated on May 16th, 2024