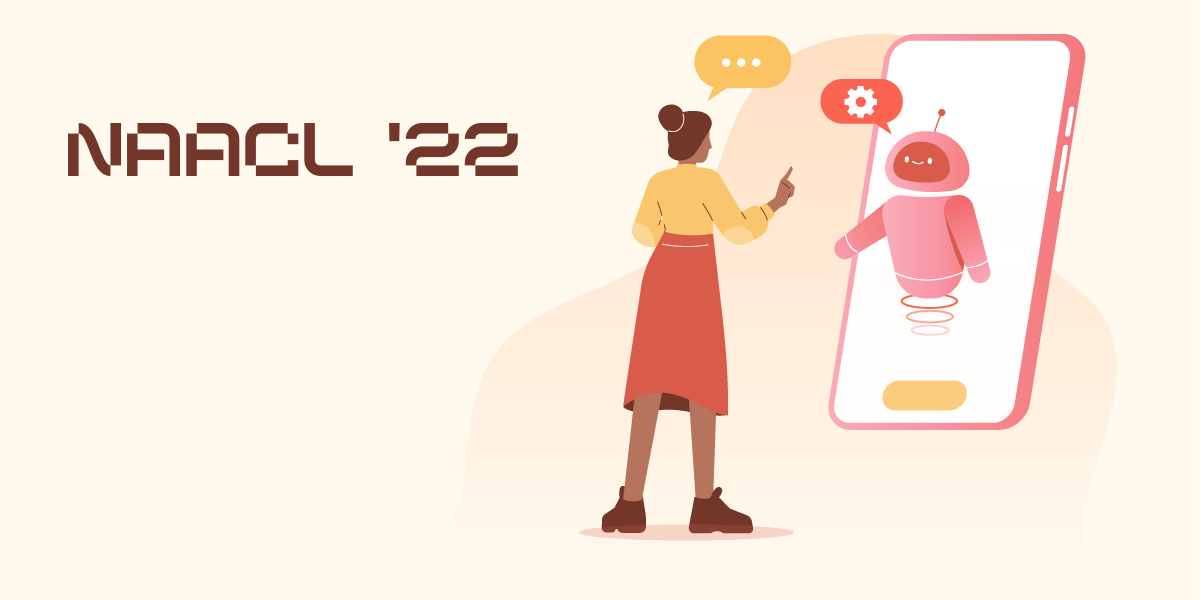
Photo credit: Muqamba/iStock
At the 2022 Annual Conference of the North American Chapter of the Association for Computational Linguistics (NAACL), held July 10-15, 2022 both online and in person in Seattle, Wash., USC’s Information Sciences Institute (ISI) researchers will present 18 studies spanning a variety of topics including reducing gender bias, avoiding toxic content creation, improving search capabilities in low-traffic e-commerce stores, using LGBTQ+ tweets to mitigate anti-queer bias and more.
Run by NAACL, which provides a regional focus for members of the Association for Computational Linguistics (ACL) in North, Central and South America, the annual conference is one of the premiere conferences for natural language research.
NAACL 2022 received a record-high 2103 submissions, topping last year’s record-high of 1797. This year, 442 papers were accepted at a rate of 21%, five percent lower than last year’s 26%.
Of the 442 accepted papers, five best papers and three outstanding papers have been announced. Among the recipients is Alexander Spangher, an ISI graduate research assistant, whose paper, “NewsEdits,” provides the first publicly available dataset of news revision histories. This paper was awarded “honorable mention for contributions to resources” for its ground-breaking work in compiling and studying newspaper article revisions.
Four affinity groups are also holding day-long workshops at the conference. In the Queer in AI workshop, ISI’s Katy Felkner, a graduate research assistant, will be presenting her paper discussing the biases against queer and trans people that are encoded in large language models and how to mitigate that bias. Felkner is one of only two researchers selected for oral presentation in the workshop.
Jonathan May, USC Viterbi research associate professor and director of ISI’s Center for Useful Techniques Enhancing Language Applications Based on Natural And Meaningful Evidence, who is co-authoring four NAACL papers this year, has served as treasurer for the organization since 2019. In this role, he has helped maintain the financial health of NAACL, while also supporting initiatives that promote the equitable expansion of research in the Americas. As chair of the Regional Americas Fund, he has been involved in giving out thousands of dollars a year to support initiatives at universities in Central and South America that promote natural language processing research. Additionally, May has helped facilitate low-cost conference child support and provide conference travel grants for NAACL conferences.
Said May, “We work toward ensuring that lack of money does not equate to lack of access.”
Pedro Szekely, USC Viterbi research professor and director of ISI’s AI division, notes that “publishing at NAACL is prestigious and provides significant visibility to the research done at ISI.”
Co-author on a paper about nuanced table-to-text generation that will be presented this year, Szekely is excited to see that the next generation of ISI researchers are having such success with papers at the conference. “We have published many papers at NAACL in our history, going back to when NAACL started. It is nice to see that our young researchers are following in the ISI tradition of pre-eminence in natural language processing.”
Research Spotlights, NAACL 2022
Avoiding Toxic Content Generation
Chatbots, dialogue systems and conversational agents, such as Siri or Alexa, interact with millions of people on a daily basis, making it increasingly important for these systems to avoid generating toxic content.
In this paper, ISI researchers studied the possibility of generating imperceptible attacks against conversational agents which, while fluent and coherent, would trigger a toxic response. Then they proposed a defense mechanism to avoid generating toxic content while keeping the conversation flowing.
According to Aram Galstyan, a USC Viterbi research associate professor and director of the Machine Intelligence and Data Science group at ISI, and his co-authors, the importance of this work is the focus on natural-looking, human-like, conversational language that might trigger a toxic response. “Such triggers might be invoked by a user during a natural conversation with a chatbot, which will result in unpleasant and offensive experiences for those unassuming users,” said Galstyan.
This differs from existing work in this area, which has largely studied attacks that do not resemble human language or are human-generated, and therefore are costly and not scalable. Galstyan and team designed a defense mechanism to mitigate attacks and maintain conversational flow. The method relies on two levels of reasoning. First, the model identifies the key adversarial tokens (i.e., words) responsible for the attack. Then, the model masks those tokens during the generation process which allows for a natural-sounding response, free from toxicity.
There are clearly mainstream applications for this work.
“We would like to see our work being incorporated into dialogue systems, such as Alexa,” said graduate research assistant and co-author, Ninareh Mehrabi. “We want to make sure these systems are robust to naturally occurring triggers.”
Using LGBTQ+ Tweets to Mitigate Anti-Queer Bias
As AI becomes increasingly ubiquitous, there is a growing concern that its algorithms are systematically biased against marginalized populations. The large majority of the research around mitigating this type of bias has been geared toward reducing race and binary gender biases.
This paper helps to fill the gap of literature dealing with queerness. Katy Felkner, graduate research assistant at ISI, said: “There was no suitable metric for measuring anti-LGBTQ+ bias in large language models, so we decided we were going to build one.” The result is WinoQueer, a new benchmark dataset modeled after other bias-detection benchmarks but addressing homophobic and transphobic biases.
Using WinoQueer to measure anti-queer bias, the researchers found that large language models show significant heteronormative bias off-the-shelf. However, when those models were fine-tuned on data about queer people, the bias was mitigated.
Using a collection of tweets from the LGBTQ+ community — which reflected the language of its members — the researchers were able to mitigate the anti-queer bias significantly. Interestingly, this performed better than the model fine-tuned on data written by mainstream news media about queer issues.
Felkner will be presenting this paper at the Queer in AI workshop at this year’s conference.
Improving the Search Capabilities of Newly Launched E-commerce Stores
Professor May and co-authors worked in partnership with Amazon to improve search systems for low-traffic e-commerce stores. Among the Amazon team was senior author Rahul Bhagat, ISI PhD alumni.
The problem is this: the systems used in e-commerce for retrieving products based on customer behavior require large amounts of customer behavior data (e.g. queries, clicks, purchases) for training. Unfortunately for low-traffic e-commerce stores, such as newly launched stores, sites or products, behavioral data is limited, impacting the performance of these systems.
“The team wanted to know if there were ways to take the small amount of customer behavior they had and reformulate it into more synthetic customer behavior. For example, if we have ‘red running shoes’ with a product page, we probably also want to associate ‘red running sneakers’ or, depending on the locale, ‘red running trainers’ with the same page,” May said.
Deep learning models can reformulate text sequences (such as “red running shoes”) into other text sequences (such as “red running sneakers” and “red running trainers”). This process is called query reformulation. In this paper, May and co-authors present a technique that uses query reformulation to augment behavioral training data of a low-traffic e-commerce store.
Using their technique, even if a particular site has less traffic, the researchers found that they could obtain millions of examples of high quality query reformulations, which could be used to train the model. “We then use the trained model to generate a lot of data,” May said.
Additionally, the researchers ran real world tests on a popular e-commerce site and found that business metrics significantly improved using their method. Two of the metrics they looked at were “search click rate” and “customer reformulation rate.” Search click rate is the percentage of searches with at least one click; more simply, it indicates how often a customer clicks on at least one of the results of their query. This metric went up using the researchers’ technique. Customer reformulation rate is the percentage of searches that a customer has to rephrase because of dissatisfaction with the initial search results, and this metric went down.
May hopes to see this technique deployed to improve customer satisfaction and reduce frustration.
Analyzing the Changing Nature of News Articles
News articles evolve over time. First, a breaking news blurb is published. This is updated as news comes in until it becomes a full-fledged article. In this paper, Spangher and co-authors present “NewsEdits,” the first publicly available dataset of news revision histories. It includes 1.2 million articles with 4.6 million versions from 22 news outlets, published over a period of eight years.
Existing research in the area of online revision histories has focused on article updates on Wikipedia, where edits are often small syntax or grammatical corrections. The research in this paper, however, shows that most news article edits incorporate new information, update events, or broaden perspectives. According to Spangher, this research shows that: “articles grow substantially over time, often by 10% or more between drafts; article updates are more likely to contain quotes, events and main-idea information; and events in these articles are likely to change and update.”
The research goes a step further, indicating that, to some extent, news article updates are predictable. “Showing that we can build models that pick up on these patterns takes us a long way to proving to the community that this dataset is modellable, and not totally crazily unpredictable,” Spangher said. The predictability shows that “this dataset is a promising candidate for modeling approaches to try to study the change processes taking place in between news articles.”
This paper is being recognized as an “honorable mention for contributions to resources” by NAACL 22, whose Best Paper Committee said, “this new resource can boost research on automatically revising articles.”
Reducing Gender Bias in AI Language Models
Artificial Intelligence is increasingly used to interact with people by generating natural language, making it ever more important to understand and mitigate the various harms that it may provoke.
“When we teach language to a computer, we use examples of things that people say in the real world,” said Research Associate Professor Greg Ver Steeg. “Unfortunately, there’s a lot of bias in the real world, and the computer learns to mimic this bias.”
The language models used in AI can be biased in multiple ways, including the unfounded association of male and female genders with gender-neutral professions. To tackle this, Ver Steeg and colleagues used counterfactual information to train their models. In other words, they automatically generated and imposed the same fact with the gender roles flipped, so that the model received equal inputs for both genders. “The system will see as many examples of ‘he is a nurse’ as it sees ‘she is a nurse,’” said Ver Steeg.
In this paper, Ver Steeg and colleagues present their approach and demonstrate that it can result in a substantial reduction in gender disparity. Additionally, Ver Steeg emphasizes the importance of this work, “Today’s methods for training artificial intelligence are focused on mathematical optimization problems which will not necessarily reflect the values of its designers. If we intend for AI to benefit society, we need to find a way to align what these systems learn with human values.”
A New and Improved Approach to Entity Typing
Assistant Research Professor Muhao Chen has an astonishing eight papers and tutorials being presented at NAACL ‘22. Among them is this paper, in which Chen and co-authors present a new approach to entity typing.
The entity typing task is a fundamental and long-lasting problem in Natural Language Processing. Co-author Bangzheng Li explains: “Given a sentence, such a task seeks to predict appropriate words or phrases to describe specific entity mentions in the context. For example, in ‘Jay is currently working on his Spring ’09 collection, which is being sponsored by the YKK Group,’ the entity ‘Jay’ should be labeled as ‘person,’ ‘designer’ or ‘creator’ instead of ‘organization’ or ‘location.’ A key challenge of this task lies in understanding the contextual information of the sentence, which is easy for human readers but complicated for machines. Our model leverages the power of natural language inference to achieve the goal.”
The researchers started with a model that was pre-trained to answer true or false questions. They then reformulated the entity typing problem as a series of true or false questions, each question asking if a candidate word or phrase described the entity well. Using this method to refine the model, the researchers achieved state-of-the-art performance on the entity typing task.
View the complete list of accepted USC ISI papers below:
StATIK: Structure and Text for Inductive Knowledge Graph Completion
Elan Sopher Markowitz, Keshav Balasubramanian, Mehrnoosh Mirtaheri, Murali Annavaram, Aram Galstyan, Greg Ver Steeg. NAACL, 2022.
Mitigating Gender Bias in Distilled Language Models via Counterfactual Role Reversal
Umang Gupta, Jwala Dhamala, Varun Kumar, Apurv Verma, Yada Pruksachatkun, Satyapriya Krishna, Rahul Gupta, Kai-Wei Chang, Greg Ver Steeg, Aram Galstyan. NAACL, 2022.
Robust Conversational Agents against Imperceptible Toxicity Triggers
Ninareh Mehrabi, Ahmad Beirami, Fred Morstatter, Aram Galstyan. NAACL, 2022.
Temporal Generalization for Spoken Language Understanding
Judith Gaspers, Anoop Kumar, Greg Ver Steeg, Aram Galstyan. NAACL, 2022.
Ultra-fine Entity Typing with Indirect Supervision from Natural Language Inference
Bangzheng Li, Wenpeng Yin, Muhao Chen. Transactions of the Association for Computational Linguistics (TACL), 2022. NAACL, 2022.
On the Robustness of Reading Comprehension Models to Entity Renaming
Jun Yan, Yang Xiao, Sagnik Mukherjee, Bill Yuchen Lin, Robin Jia, Xiang Ren. NAACL, 2022.
Unified Semantic Typing with Meaningful Label Inference
James Y. Huang, Bangzheng Li, Jiashu Xu, Muhao Chen. NAACL, 2022.
Should We Rely on Entity Mentions for Relation Extraction? Debiasing Relation Extraction with Counterfactual Analysis
Yiwei Wang, Muhao Chen, Wenxuan Zhou, Yujun Cai, Yuxuan Liang, Dayiheng Liu, Baosong Yang, Juncheng Liu, Bryan Hooi. NAACL, 2022.
Robust (Controlled) Table-to-Text Generation with Structure-Aware Equivariance Learning
Fei Wang, Zhewei Xu, Pedro Szekely, Muhao Chen. NAACL, 2022.
Answer Consolidation: Formulation and Benchmarking
Wenxuan Zhou, Qiang Ning, Heba Elfardy, Kevin Small, Muhao Chen. NAACL, 2022.
DEGREE: A Data-efficient Generation-based Event Extraction Model
I-Hung Hsu, Kuan-Hao Huang, Elizabeth Boschee, Scott Miller, Prem Natarajan, Kai-Wei Chang, Nanyun Peng. NAACL, 2022.
Augmenting Training Data for Massive Semantic Matching Models in Low-Traffic E-commerce Stores
Ashutosh Joshi, Shankar Vishwanath, Choon Hui Teo, Vaclav Petricek, Vishy Vishwanathan, Rahul Bhagat, Jonathan May. NAACL Industry Track, 2022.
NewsEdits: A News Article Revision Dataset and a Novel Document-Level Reasoning Challenge
Alexander Spangher, Xiang Ren, Jonathan May, Nanyun Peng. NAACL, 2022.
New Frontiers of Information Extraction
Muhao Chen, Lifu Huang, Manling Li, Ben Zhou, Heng Ji, Dan Roth. Tutorial at NAACL, 2022.
Dangling-Aware Entity Alignment with Mixed High-Order Proximities
Juncheng Liu, Zequn Sun, Bryan Hooi, Yiwei Wang, Dayiheng Liu, Baosong Yang, Xiaokui Xiao, Muhao Chen. Findings of NAACL, 2022.
GraphCache: Message Passing as Caching for Sentence-Level Relation Extraction
Yiwei Wang, Muhao Chen, Wenxuan Zhou, Yujun Cai, Yuxuan Liang, Bryan Hooi. Findings of NAACL, 2022.
Towards WinoQueer: Developing a Benchmark for Anti-Queer Bias in Large Language Models
Virginia K. Felkner, Eugene Jang, Ho-Chun Herbert Chang, Jonathan May. Queer in AI Workshop at NAACL, 2022.
Published on July 11th, 2022
Last updated on May 16th, 2024