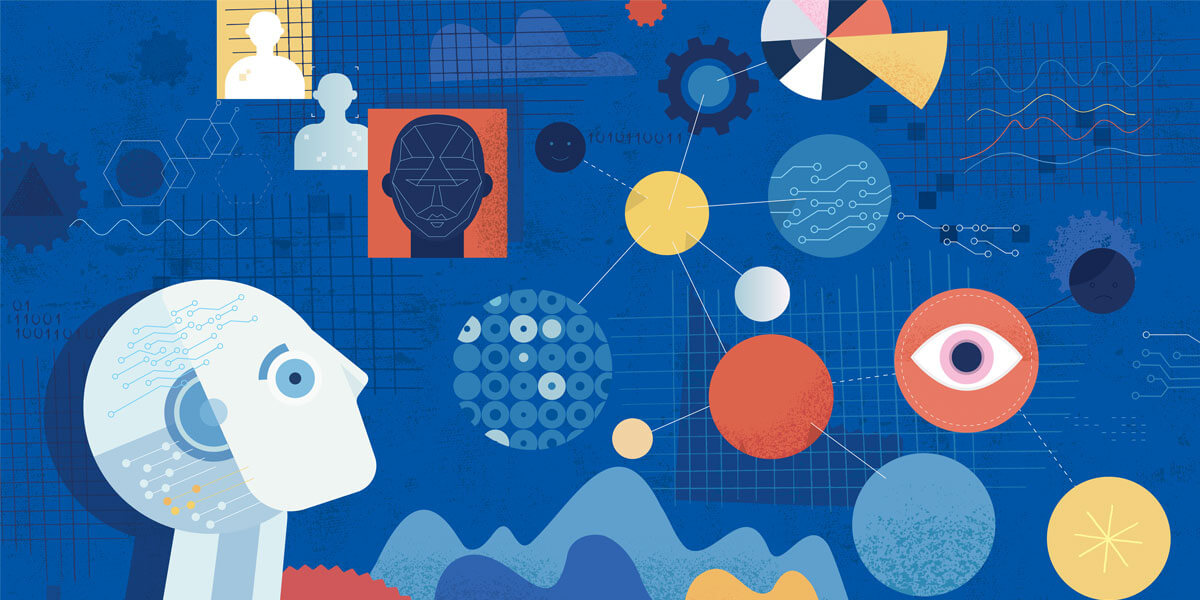
RoboCLIP dramatically reduces how much data is needed to train robots, allowing anyone to interact with them through language or video. Photo/iStock.
Alone at home, your bones creaky due to old age, you crave a cool beverage.
You turn to your robot and say, “Please get me a tall glass of water from the refrigerator.”
Your AI-trained companion obliges.
Soon, your thirst is quenched.
While this scenario still is a decade or more away in terms of a seamless real-world application, a new research paper led by USC computer science student Sumedh A. Sontakke with his advisors Assistant Professor Erdem Bıyık and Professor Laurent Itti, opens the door wider to this potential reality with a new online algorithm they created called RoboCLIP.
“The most impressive thing about RoboCLIP is being able to make our robots do something based on only one video demonstration or one language description.” Erdem Biyik
Aging populations and caregivers stand to benefit the most from future work based on RoboCLIP, which dramatically reduces how much data is needed to train robots by allowing anyone to interact with them through language or videos – at least, for now, in computer simulations.
“To me, the most impressive thing about RoboCLIP is being able to make our robots do something based on only one video demonstration or one language description,” says Biyik, a roboticist who joined USC Viterbi’s Thomas Lord Department of Computer Science in August 2023 and leads the Learning and Interactive Robot Autonomy Lab (Lira Lab).
Learning quickly with few demonstrations
The paper, titled RoboCLIP: One Demonstration is Enough to Learn Robot Policies, will be presented by Sontakke at the 37th Conference on Neural Information Processing Systems (NeurIPS), Dec. 10-16 in New Orleans.
“The large amount of data currently required to get a robot to successfully do the task you want it to do is not feasible in the real world, where you want robots that can learn quickly with few demonstrations,” Sontakke explains.
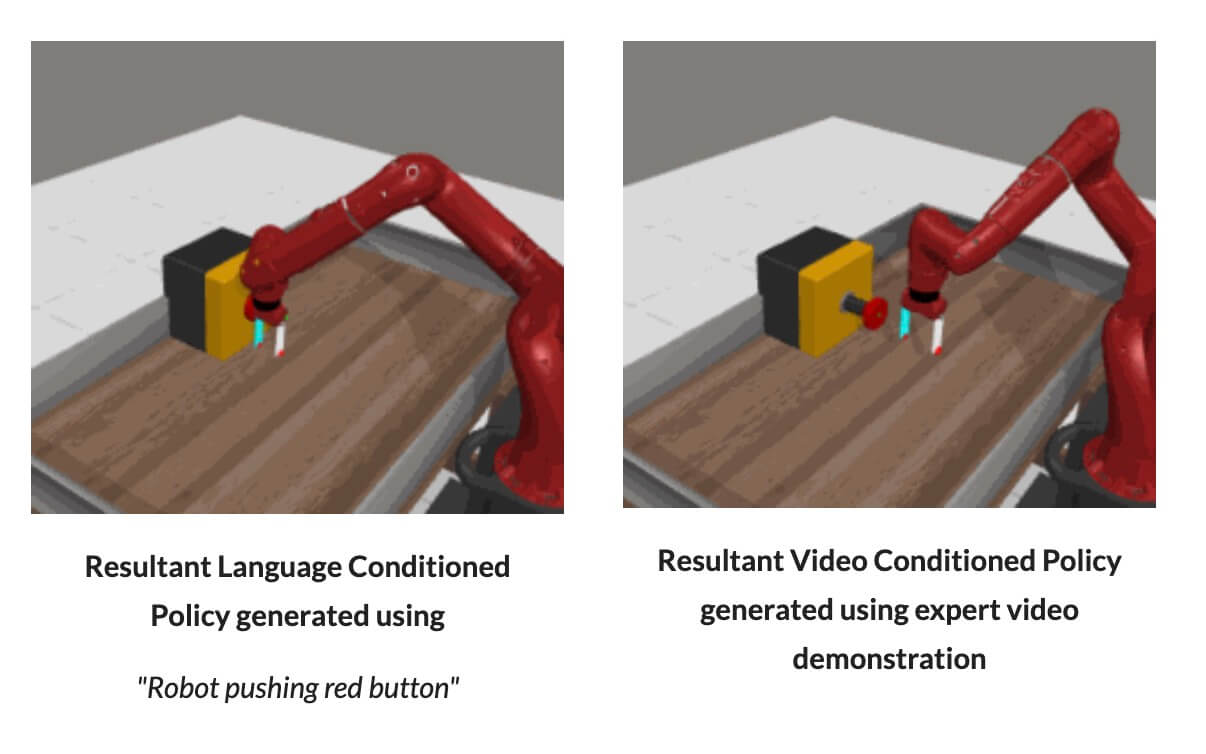
Using only one video or textual demonstration of a task, RoboCLIP performed two to three times better than other imitation learning (IL) methods.
To get around this notoriously difficult problem in reinforcement learning—a subset of AI in which a machine learns by trial and error how to behave to get the best reward—the researchers tested RoboCLIP.
Result?
Using only one video or textual demonstration of a task, RoboCLIP performed two to three times better than other imitation learning (IL) methods.
Future research is needed before this study translates into a world where robots can learn quickly with few demonstrations or instructions – such as fetching you a tall glass of chilled water – but RoboCLIP represents a significant step forward in IL research, Sontakke and Biyik said.
Right now, IL methods require many demonstrations, massive datasets, and substantial human supervision for a robot to master a task in computer simulations.
Now it can learn from just one, the RoboCLIP research shows.
Performing well “out of the box”
RoboCLIP was inspired by advances in the field of generative AI and video-language models (VLMs), which are pretrained on large amounts of video and textual demonstrations, Sontakke and Biyik explained. The new algorithm harnesses the power of these VLM embeddings to train robots.
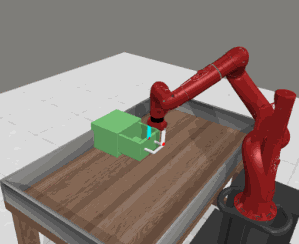
In simulation, the robot successfully closes the drawer with a single video demonstration or text description.
A handful of experimental videos on the RoboCLIP website show the method’s effectiveness.
In the videos, a robot – in computer simulations — pushes a red button, closes a black box, and closes a green drawer after being instructed with a single video demonstration or a textual description (for example, “Robot pushing red button”).
“Out of the box,” Biyik says, “RoboCLIP has performed well.”
Two years in the making
Sontakke said the genesis of the research paper dates back two years ago.
“I started thinking about household tasks like opening doors and cabinets,” he said. “I didn’t like how much data I needed to collect before I could get the robot to successfully do the task I cared about. I wanted to avoid that, and that’s where this project came from.”
Collaborating with Sontakke, Biyik and Itti on the RoboCLIP paper were two USC Viterbi graduates, Sebastien M.R. Arnold, now at Google Research, and Karl Pertsch, now at UC Berkeley and Stanford University. Jesse Zhang, a fourth-year Ph.D. candidate in computer sciences at USC Viterbi, also worked on the RoboCLIP project.
‘Key innovation’
“The key innovation here is using the VLM to critically ‘observe’ simulations of the virtual robot babbling around while trying to perform the task, until at some point it starts getting it right – at that point, the VLM will recognize that progress and reward the virtual robot to keep trying in this direction,” Itti explained.
“This new kind of closed-loop interaction is very exciting to me.” Laurent Itti
“The VLM can recognize that the virtual robot is getting closer to success when the textual description produced by the VLM observing the robot motions becomes closer to what the user wants,” Itti added. “This new kind of closed-loop interaction is very exciting to me and will likely have many more future applications in other domains.”
Besides the aging population who will rely on robots to improve their daily lives, RoboCLIP could lead to applications that could help anyone.
Think of those DIY videos you look up on YouTube to figure out how to fix a busted garbage disposal or malfunctioning microwave.
Could you simply, in the future, ask your robot assistant to perform such tasks while you slumber on the couch?
The possibilities are intriguing, Biyik and Sontakke said.
Published on December 11th, 2023
Last updated on May 16th, 2024