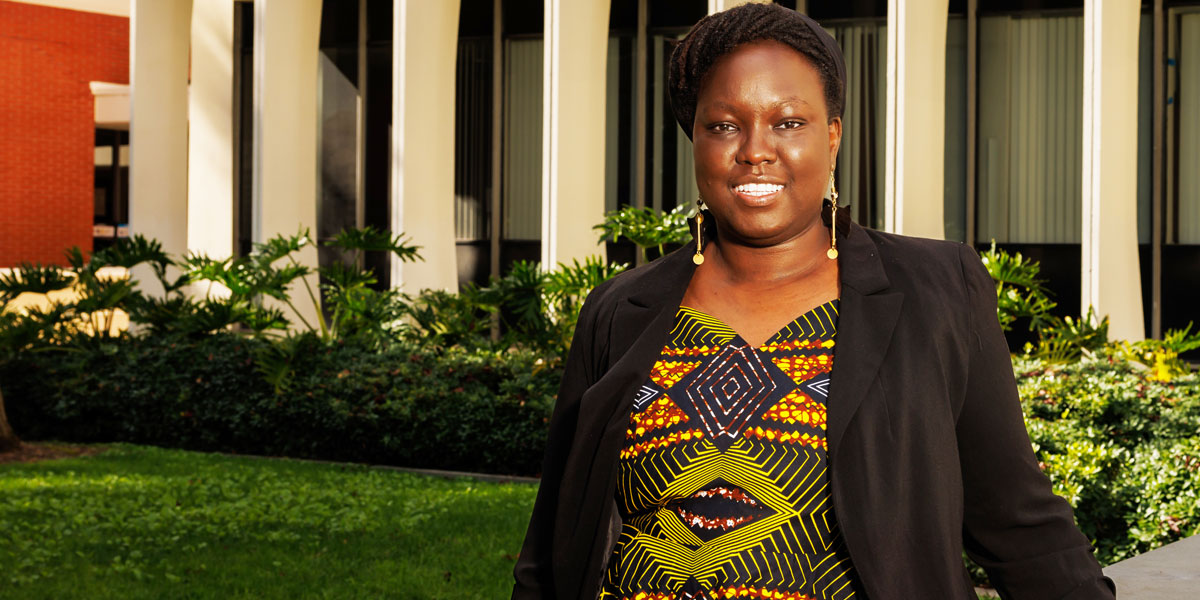
Doctoral student Elizabeth Ondula is applying AI, specifically reinforcement learning algorithms, to manage in-person attendance during epidemics. Photo/Helen Perez.
The first time Elizabeth Ondula thought about using technology to improve public health was when she faced a personal tragedy during the COVID-19 pandemic. Her father died and she was unable to travel for his burial due to pandemic restrictions. “It was a challenging time,” said Ondula. “The travel restrictions made me wonder why public health policies are static, and how these strategies could be more adaptable to evolving conditions.”
Now a doctoral student in computer science at USC, Ondula’s research examines how policymakers and administrators can leverage AI to prepare for an infectious disease outbreak. On Feb. 21, Ondula will present her research on “Navigating Uncertainty in Epidemic Contexts with Reinforcement Learning” at the Association for the Advancement of Artificial Intelligence (AAAI) doctoral consortium.
“What this research brings to the table is an understanding of the impact of various policies,” said Ondula. “If you prepare and plan well, you can minimize the risk of serious illness or death, while responding to rapidly evolving conditions.”
Ondula’s application of AI, specifically reinforcement learning algorithms, to manage in-person attendance during epidemics offers “an important contribution at the intersection of public health, education, and artificial intelligence,” said her advisor and co-author Bhaskar Krishnamachari, Ming Hsieh Faculty Fellow in Electrical and Computer Engineering-Systems and Professor of Electrical and Computer Engineering and Computer Science.
Specifically, Ondula believes the tool could lead to faster and better implementation of hybrid policies at school, work, and for travel, and help hospitals manage capacity issues due to surges. By running theoretical scenarios, the tool could help policymakers and administrators better understand the impact of various possible decisions to find one that is “just right.”
An early warning system
Ondula’s technology aims to determine how many people can safely access a public space, such as an office or a learning facility, during an epidemic.
During COVID-19, school closures took 50 million children out of classrooms, erasing more than 20 years of progress on national test results according to a McKinsey and Company report, a problem which is even more pronounced in poorer districts.
But how many students could have attended in-person classes at a minimal infection risk? Ondula’s work focuses on how environmental factors influence the answer to this question.
She is currently using stochastic epidemic models–mathematical models that simulate the spread of infectious diseases–and reinforcement learning techniques–a type of machine learning–to map potential scenarios for a school semester. For example, Ondula’s AI could use real-time data to act as an early warning system and recommend when to limit building occupancy.
While epidemic models consider all predictable ways a disease can spread under certain conditions, such as transmission and immunity rate, stochastic models additionally factor in how people get sick by chance. This makes them particularly well-suited to predicting real-world scenarios, according to Ondula.
“After all, to some extent, we still don’t understand how the spread of disease is dynamic,” she said. “How the disease progresses is also to some extent unknown. This model helps to capture that randomness that you’re dealing with in real-world scenarios.”
An important benefit of her concept is its applicability to any closed space. During COVID-19, for instance, hospitals hitting capacity needed to maximize space occupancy without knowing how their decisions could compromise patient safety. Ondula’s technology could provide an answer.
Moreover, the software can explore other epidemics, such as dengue fever, which spreads through mosquito bites. Ondula hopes to make the technology open source by May 2024, allowing researchers to run simulations with all kinds of models and settings. Next, Ondula will interview school administrators and policymakers to understand and resolve potential implementation issues.
“By integrating stochastic epidemic models with reinforcement learning, Elizabeth’s work offers a novel approach to developing a data-driven decision aid tool for campus administrators that can suggest a good tradeoff between health concerns and learning objectives,” said Krishnamachari. “Her research not only advances academic understanding but also has practical implications for enhancing epidemic response strategies in the future.”
Published on February 22nd, 2024
Last updated on May 16th, 2024