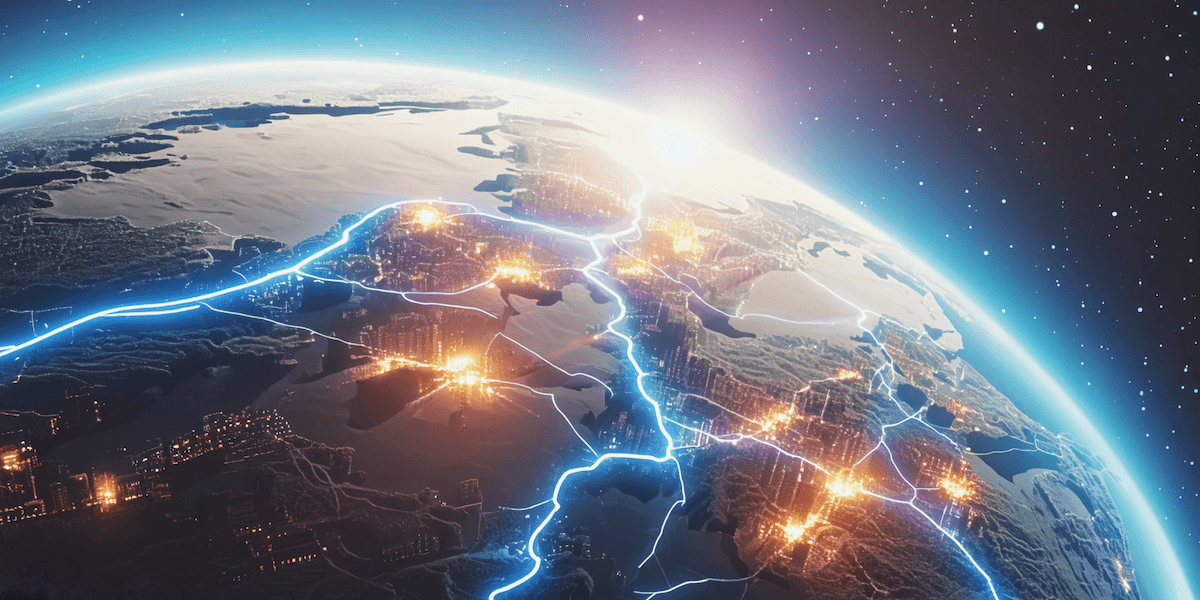
A rendering that shows the demand for energy by the world’s metropolises/Image credit: Runway ML
The growth and impact of artificial intelligence are limited by the power and energy that it takes to train machine learning models. So how are researchers at the USC School of Advanced Computing and other units within the USC Viterbi School of Engineering working to improve computing efficiency to support the rising demand for AI and its requisite computing power?
According to Murali Annavaram, the Lloyd F. Hunt Chair of Electrical Power Engineering and Professor of Electrical and Computing Engineering and Computer Science, when we teach computers to learn, there are three factors that affect how much time and energy the learning takes: how big the model is; how big the dataset is or how much information the computer needs to process; and the efficiency of the computer that conducts these tasks.
Researchers within the USC School of Advanced Computing have taken different approaches to make AI computing more efficient. Some are trying to make the AI algorithms, which require a lot of energy, less demanding. Others are trying to change the design and functionality of the computers chips that process information, including the very materials of which the chips are made.
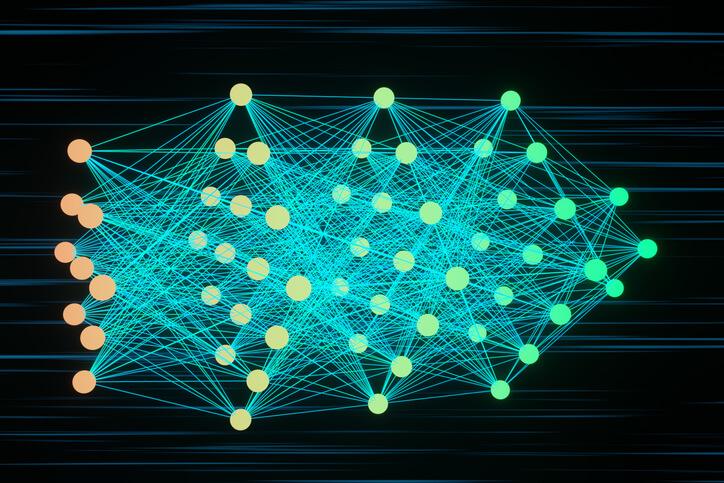
Illustration of the concept of deep neural network and generative artificial intelligence/Image credit: Dragon Claws for iStock
Changing the Way AI Learns:
When we learn a new word as infants, such as the word ‘‘dog,’ we might overgeneralize at first, calling every four-legged animal a dog. But we don’t need to see every dog in the world to recognize one. Our brains form patterns based on limited experience and refine them over time.
Machines, on the other hand, learn through neural networks that identify patterns in data and draw conclusions. Neural networks inspired by biological networks of neurons such as those in human brains, function as a vast, interconnected web, cycling through billions of training samples to learn the relationships and extract meaning from new information. For instance, modern AI training models may cycle through millions of different animal images that are explicitly labeled in order to learn to later recognize an unlabeled four-legged creature based on information on which it was previously trained. Similarly, models like ChatGPT cycle through billions of pages of text on the Internet to become adept at text-completion tasks.
But this machine-driven scavenger hunt demands enormous amounts of energy. Massoud Pedram, the Charles Lee Powell Chair in Electrical and Computer Engineering and Computer Science and Professor of Electrical and Computer Engineering, asks a critical question: can we teach AI to learn more effectively using fewer steps or examples?
One approach used by Pedram’s lab is to simplify machine learning models. Using methods like pruning, his team “trims” off the unnecessary parts of the neural networks, like cutting dead branches on a plant. This shortens the number of steps needed by narrowing the parameters and possibilities, making AI learning faster and more energy efficient.
His team also develops methods for quantization, which use fewer bits to represent data and model parameters. Additionally, his team leverages pre-trained knowledge and transfers learning from related tasks to facilitate efficient learning with limited data. Pedram notes that this approach “results in shorter training times, reduced hardware resource requirements, higher energy efficiency, and improved generalization, ultimately ensuring better performance on new, related tasks at a lower computational cost.”
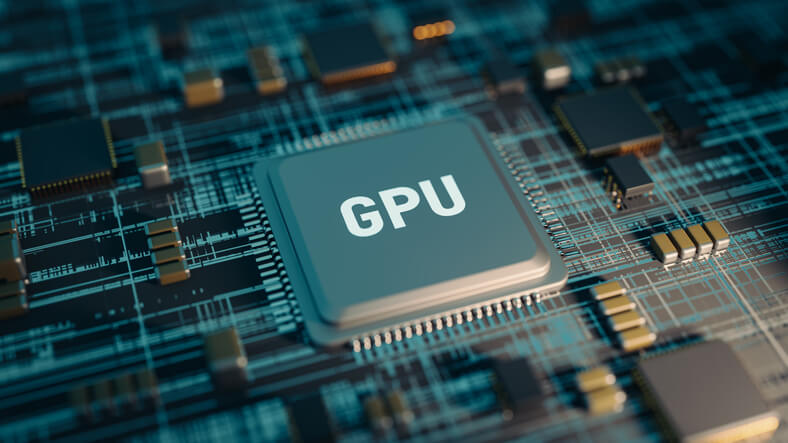
Rendering of GPU computing chip of graphics card/Image credit: Mesh Cube for istock
Changing the Type of Hardware: The Case for Using CPUs versus GPUS for AI and Large Language Models:
Professor Annavaram says that for a long time, the industry focused a lot on making machine learning models and datasets bigger. This is because the larger the datasets, the more information and examples you have, and the more likely your conclusions generated by AI will be accurate. This move towards larger and larger models led to the creation of a new era of machine learning models called Large Language Models (LLMs). Nowadays, LLMs can complete tasks such as summarizing all the user reviews for a given restaurant and automatically generating a star rating for the restaurant based on the “sentiment” extracted from its reviews.
But “focusing on model performance without efficiency considerations will hit an unsustainable climb on the cost wall,” says Annavaram.
Currently, AI often relies on specialized hardware: devices called graphics processing units or “GPUs” for short. They were developed for graphics but have been found to excel for AI. However, says Annavaram, they are incredibly expensive and energy-intensive.
Instead of using solely GPUs for large language models, Annavaram’s team is relying on less expensive computers known as CPUs or (central processing units) to help with some “pre-processing” of data. More specifically, his lab is advocating for using the much cheaper and plentiful CPU memory to reduce the cost of using expensive GPU memory. He suggests combining the advantages of both CPUs and GPUs: using CPU memory for storing the model information while using GPUs to perform computations. With this approach, Annavaram says, the overall system efficiency can be improved.
In another approach, the Pedram Lab specializes in custom hardware optimization, optimizing machine learning models for specific hardware platforms, such as specialized chips, graphic processing units, and field programmable gate array devices, and fine-tuning pre-trained machine learning models for specific tasks, which Pedram indicates yields significant energy efficiency gains. One can liken this to using different knives for different chopping tasks or different types of bicycle tires for different terrains.
Pedram also optimizes how data, which feeds AI/ML model training and inference, is input into devices along with data storage, which he notes drains time and energy.

Empty cockpit of autonomous car, HUD(Head Up Display) and digital speedometer. self-driving vehicle/Image credit: Stock.
Where computing is processed: Living at the Edge
Over a decade ago, NYC investment banks were investing in data centers to get information a fraction of a second faster than peers and allowing for more profits from high-frequency trading. Edge computing has a similar premise—allowing for the processing of information closer to the action where the data is received versus at centralized data centers.
“Processing information ‘at the edge’ can significantly reduce energy consumption,” says Pedram. One context in which edge computing technology could be particularly useful is for driverless cars that need to process information about road conditions immediately for instantaneous decision-making.
Pedram says, “This emerging technology, which could perform most of the AI/ML workload processing on the edge, enables faster responses, conserves bandwidth, enhances data privacy, and improves scalability.”
Information “Batteries”
Associate Professor of Computer Science Barath Raghavan is wondering if smaller steps could yield big energy savings. Imagine, says Barath, if computer users could do energy-intensive pre-computations for AI when energy is not in high demand, thus lessening the demand on the grid. That’s the idea behind his notion of “information batteries,” whereby computations are completed in advance versus all at once.
“Information batteries could both save energy and help the grid as a means of energy storage,” says Raghavan.
“Right now, there are times of the year when there is too much solar energy, energy that would literally be dumped if not used. Time-shifted speculative computation can use that energy productively. By storing the output of that computation, this stores energy in the form of useful computation results, enabling us to soak up power from the grid when it’s in surplus and use it when it’s not,” he adds.
Changing How Computers Are Designed Part 1: Superconducting Electronics
“Over the last decade, power and energy budgets have become limiting factors for complementary metal-oxide semiconductor (known as CMOS) chips due to the increasing cost of power distribution as well as the cost of keeping computer chips cool,” says Peter Beerel, Professor of Electrical and Computer Engineering.
Beerel says, “A radical approach to address this problem is what’s known as superconducting electronics that promise 100x to 1,000x lower power than CMOS with the same, if not higher, performance.”
“It calls for a new paradigm to rebuild and design computers,” says Beerel.
As of now, computers rely on semiconductors or chips made of silicon, which include switches called transistors. These transistors are made from semiconductors, which take advantage of their property to conduct a charge only when properly stimulated. This partial conducting allows for the binary code “0’s” (the “off” switch) and 1’s ( the “on” switch) to underpin all CMOS computer chips. But this switching back and forth consumes and wastes energy. So for the last decade, Beerel’s lab has been trying to make computers work in a different fashion via superconductors—a method that uses a new conducting material niobium that doesn’t have resistance nor a switch-like transistor, but a loop called a Josephson Junction.
To generate the “0” or the “off” button the charge works in one direction of a loop, for an “on” button, it flows in another direction of the same loop.
In particular, Beerel’s lab has recently developed algorithms that control or “clock” data through the different parts of a chip to help deliver high-performance with ultra-low energy consumption.
Collaborating with Professor Massoud Pedram and his group, Beerel has also begun a third project to extend his research to cover what he says “promises similar low power benefits with higher design densities.”
As this new technology evolves, his group hopes to “continue to develop new software tools that will help automate design and enable superconducting technology to scale.”
Novel Materials
Jay Ravichandran, the Philip and Cayley MacDonald Endowed Early Career Chair and Professor of Chemical Engineering and Materials Science and Electrical and Computer Engineering, is synthesizing new materials for energy-efficient computing. Like Beerel, he notes the inefficiencies of current materials involved in semiconducting.
He says, “The major theme of our work is to realize abrupt switching materials (such as oxides and chalcogenides) and devices that require a small external impulse such as voltage bias, or temperature increase to create dramatic change in the electrical properties.”
“In addition to their potential for energy-efficient computing, these materials hold significant promise for neuromorphic computing, which seeks to mimic the way biological systems, specifically the brain, process information,” says Ravichandran.
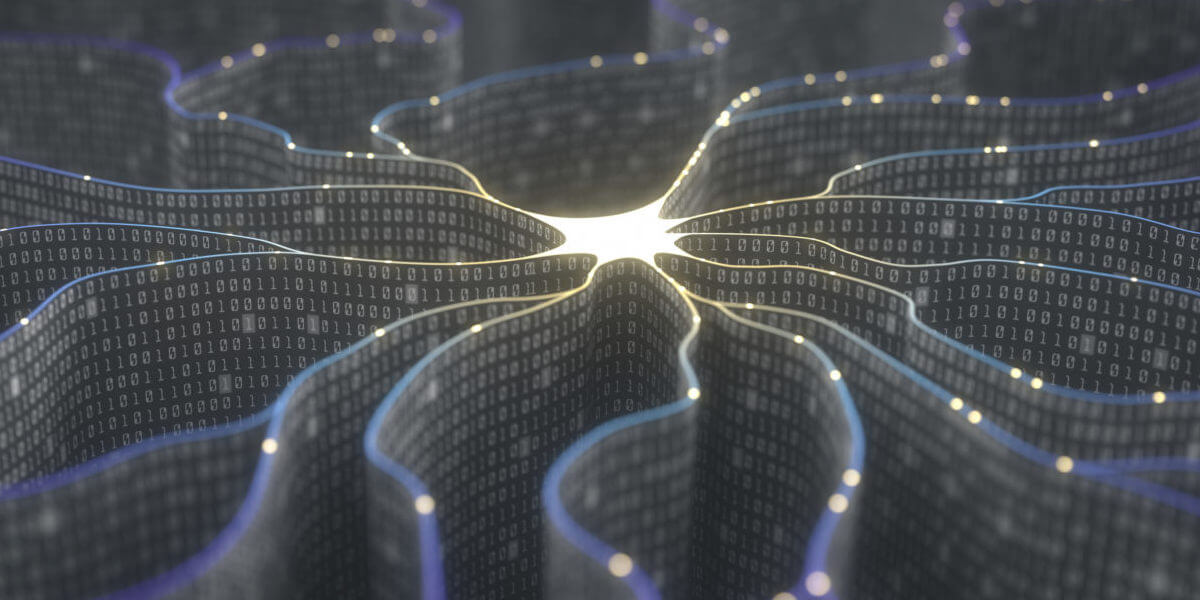
Artificial neural network and data transmission (Photo credit/iStock)
Copying the Human Brain
While computers might do things faster than humans, they are not as efficient. “The human brain is efficient,”Pedram says.
He adds, “It consumes approximately 0.3 kilowatt hours daily, primarily through metabolic processes. In contrast, the average graphic processing unit (GPU) consumes about 10 to 15 kilowatt hours daily.”
“This significant disparity highlights the potential of neuromorphic computing: developing models and hardware that mimic the brain’s efficient operation,” says Professor Pedram. His lab has been “exploring novel approaches in neuromorphic computing, including biologically-inspired neuron and synapse models, and hybrid networks that combine oscillators and spiking neurons.”
At USC, neuromorphic computing really got its start from Emeritus ECE professor and neuromorphic computing pioneer Alice Parker, who came to USC in 1980. While Parker was trying to mimic the brain’s circuitry to address mental health challenges, other scholars continue her legacy to ensure that computing is more energy efficient.
Now, it is taken to the next level by Joshua Yang, the Arthur B. Freeman Chair Professor of Electrical and Computer Engineering, who leads a U.S. Airforce Center of Excellence based on his work on neuromorphic computing.
The Center of Neuromorphic Computing Under Extreme Environment, or CONCRETE, convenes scholars from various universities. Yang, as a principal scholar, has developed innovations and has a body of research that contribute to the Center’s thought leadership, including developing devices that work differently than devices seen in traditional computers.
While traditional computers separate memory and processing, Yang developed metal oxide memristors, which are electronic devices the size of one-billionth of a meter (a nanoscale) that act like synapses in the brain, co-locating data storage and processing in the same location. In addition to processing and storing in the same location, the data is also stored in an analog format which is more compatible with real-world data and not in the 0’s and 1’s of digital data storage. Without the step of converting real-world data into a digital format, Yang’s next-generation “analog in-memory” computing chips drastically reduce power consumption while enhancing processing speed.
While preserving energy efficiency and processing speed, Yang’s recent work focuses on addressing the primary drawback of analog computing—its relatively low precision. His group has achieved the highest precision per memory unit and demonstrated precision at the chip level through an innovative design that integrates these devices. He notes, “These innovations significantly improve memory precision and efficiency, a crucial advancement for AI and neuromorphic computing.”
This work is not just conceptual. It’s happening in reality; Yang also has his own startup, Tetramem, which is cultivating the technology for use in new electronic devices.
Published on April 28th, 2025
Last updated on April 29th, 2025