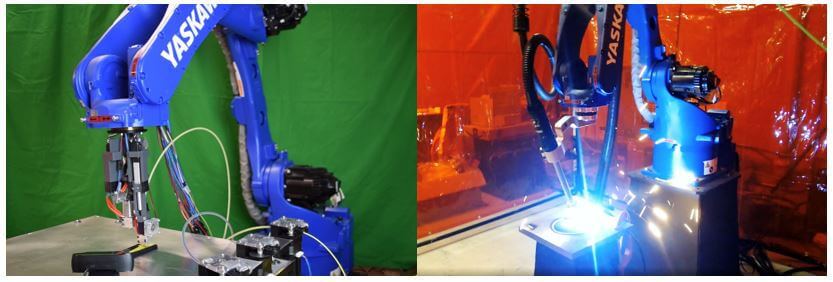
Robotic manufacturing processes which require high trajectory execution accuracy. (Left) robotic additive manufacturing and (right) robotic welding.(PHOTO/SK Gupta)
The top two best research papers at the American Society of Mechanical Engineer’s annual Manufacturing Science and Engineering Conference (MSEC) belonged to students from the USC Viterbi School of Engineering.
This year’s conference, held virtually over June 21 to 25 due to COVID-19, saw 168 submissions from students hailing from institutions all over the country. Despite the rigorous competition, the common denominator among the best was USC Viterbi’s Center for Advanced Manufacturing (CAM), directed by Professor Satyandra K. Gupta.
“Under Professor SK Gupta’s visionary leadership, in strong partnership with exceptional innovators such as Professor [Yong] Chen, and in a short period of time, CAM has become a thought leader,” said USC Viterbi Dean Yannis Yortsos. “In this vibrant research and innovation ecosystem they’ve created, CAM continues to make great advances in the rapidly evolving advanced manufacturing area. We are very proud of their efforts.”
First Place:
“Hybrid-Light-Source Stereolithography For Fabricating Macro-Objects With Micro-Textures”
Co-authors: Wenxuan Jia, Yuen-Shan Leung, Huachao Mao, Han Xu, Chi Zhou
Advisor: Yong Chen
Inspired by the complex, yet effective surface structures that occur in nature, these co-authors — a team comprised of Jia, a former USC Viterbi undergraduate; Xu, a current USC Viterbi Ph.D. student; Leung, Mao, and Zhou, former USC Viterbi Ph.D. students — studied ways to mimic these macro-bodied, micro-textured structures.
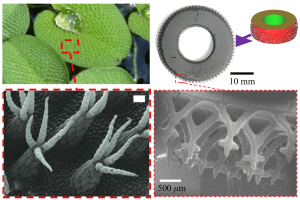
Functional micro-features found on bodies of creatures and 3D printed parts. (PHOTO/Yong Chen)
In traditional manufacturing processes like milling, it’s difficult to fabricate large objects (macro-bodies) that possess complex surface textures (micro-textures). This is because macro-bodies appear smooth at a glance, but when examined closely, have functional micro features. For example, the multicellular hairs that act as scales or glands on the smooth surface of a leaf.
The top ranked paper demonstrates the feasibility of fabricating large structures with micro-features as small as 50 micrometers, and at incredibly high throughput and complex shape control.
“While the applications of these findings require more research, it’s my prediction that a decade from now, anyone will be able to 3-D print complex structures with texture detail down to the micrometers,” said Chen, USC Viterbi professor of industrial and systems engineering and aerospace and mechanical engineering. “This will be particularly useful in fields like large medical tools or devices that require extreme texture precision in order to interact with cells and delicate human bodies.”
Jia, a current Ph.D. student at the Massachusetts Institute of Technology, was a fourth-year undergraduate student at USC Viterbi while he was performing research related to the award.
Said Chen, “It’s extremely rare for a Ph.D. student, let alone an undergraduate to be awarded a best paper award in a major research conference. We’re extremely proud of our students.”
Second Place:
“Trajectory-Dependent Compensation Scheme To Reduce Manipulator Execution Errors For Manufacturing Applications”
Co-authors: Prahar M. Bhatt, Rishi K. Malhan, Pradeep Rajendran, and Aniruddha V. Shembekar
Advisor: Satyandra K. Gupta
Improving accuracy of robotic manipulation was the theme of this paper which ranked second overall in the competition.
In the manufacturing world, robots are often used to perform a wide variety of manufacturing processes that require high trajectory execution accuracy or, in other words, near perfect movement along a path to achieve a certain outcome. When these processes are given automatically generated trajectories, they often exhibit significant execution errors to due to robot inaccuracies and controller behavior.
The second-ranked paper proposed a method for robots to curb these execution errors by sampling input trajectories, generating their own training data by measuring these samples, and learning a compensation scheme to modify further input trajectories from their findings.
Rather than humans repeating failed experiments caused by the uncertainty of automated trajectories, this advanced machine learning technique allows robots to become “self-aware” of their movements and learn from their mistakes.
“If I only have two hours to complete a task, the last thing I want is to spend that time deciphering errors and compensating the whole task,” said Bhatt, first co-author on the paper, “but rather have a robot spend two minutes learning from the errors quickly and compensating for said errors directly.”
These findings—smart, speedy robots that keep production levels high—are especially relevant for fast-paced manufacturing industries that demand immense precision.
Said Bhatt, “We’ve designed a scheme in such a way that these incredibly large robots operate reliably and robustly while maintaining high accuracy and not to harm humans with any sudden movements. That’s where the novelty in our research lies.”
The projects conducted by each of these research groups were partially funded by the National Science Foundation.
“We were thrilled to see our students rank so highly in such a competitive landscape,” said Gupta, the Smith International Professor of Mechanical Engineering and Computer Science. “It accurately reflects the effort and passion they give to their work, and they deserve such notable recognition.”
Published on August 31st, 2021
Last updated on August 31st, 2021