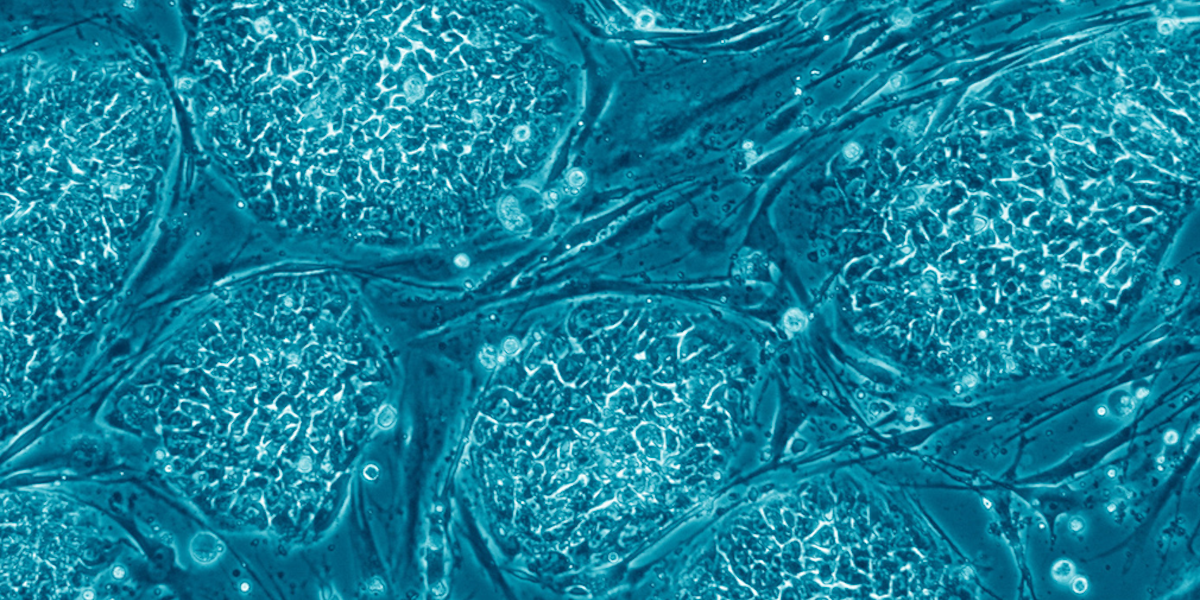
Human stem cells. Image/Wikimedia Commons
Stem cells are like the emergency tool kit of the human body. They have the unique ability to form into other types of specialized cells – from immune cells to brain cells. They can divide and regenerate indefinitely to repair and replenish our system on command.
The ability to culture stem cells in the lab and grow them into any type of cell we need is the Holy Grail of medicine. This ability could, for instance, enable clinicians to create an endless stock of new cells for repairing damaged tissues and organs. However, to unearth that Holy Grail, we need a comprehensive understanding of how stem cells replicate and transition into different cell types.
New research from USC’s Alfred E. Mann Department of Biomedical Engineering brings us one step closer to unraveling the mysteries of these essential cells. Associate Professor of Biomedical Engineering Keyue Shen and his team have harnessed machine learning to develop a non-invasive system that offers an unseen insight into how stem cells proliferate and regenerate into specialized cells. The work has been published in Science Advances.
Shen said that stem cell behavior is still quite mysterious, and the process of understanding how they divide and change is often invasive, requiring stem cells to be extracted and ultimately destroyed in the laboratory.
The new work examines the hematopoietic stem cells, which live in our bone marrow and give rise to all the cells in our blood, such as red blood cells and immune cells. For the stem cells to expand their population, Shen said they need to divide symmetrically, and for them to renew themselves while creating a new, different cell type (such as a red or white blood cell), they need to divide asymmetrically.
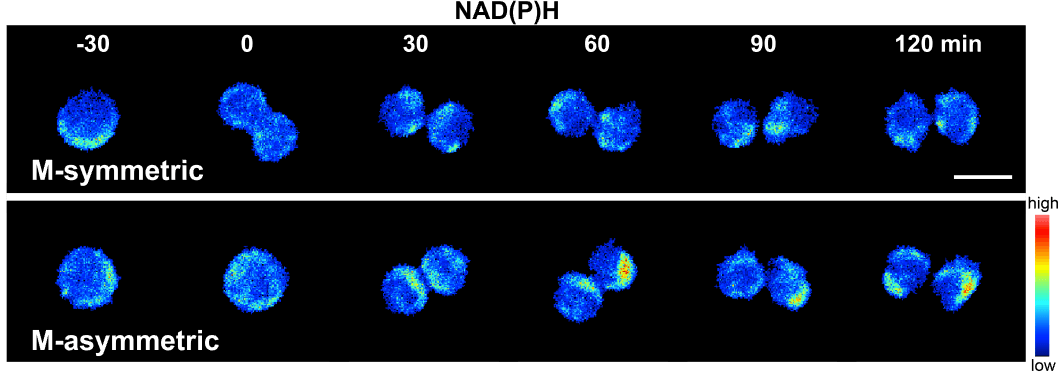
Single hematopoietic stem cells dividing metabolically (M) symmetrically and asymmetrically, measured by the research team’s imaging technique. Image/Hao Zhou and Keyue Shen
“In the case of bone marrow transplants, we want the stem cells to divide symmetrically to give us as many stem cells as possible so that we can use them on different patients. But right now, the blood stem cells cannot really be expanded outside a body in the clinic,” Shen said. “If we can achieve that – to make a huge stock of hematopoietic stem cells for bone marrow transplantation – it’s going to solve a very big problem for a lot of patients.”
Shen’s team looked at the stem cell’s metabolic behavior – how it breaks down glucose into energy using a real-time imaging technology known as fluorescence lifetime imaging microscopy.
The stem cells produce their own fluorescent material – known as autofluorescence – allowing the imaging to track the metabolism of the cells. This metabolism is strongly connected to how the cells will function and transition.
“For example, NADH is one of these molecules that’s autofluorescent and when they bond to a metabolic enzyme, they also show different optical fluorescent properties that we can measure. So in this way, we can measure them non-invasively without killing the cells,” Shen said.
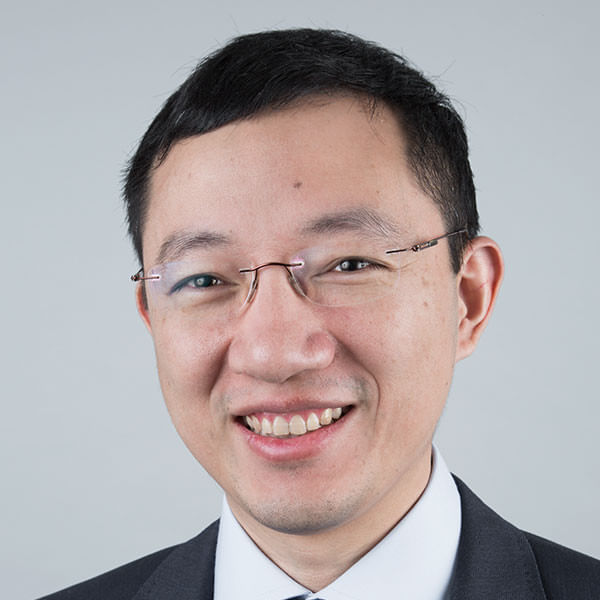
Assistant Professor Keyue Shen. Photo credit/Viterbi Staff
Using a mouse model, Shen and his team took this information and extracted the fluorescent features from stem cell images, developing a library of 205 metabolic optical biomarker features from each individual stem cell, 56 of which were associated with the differentiation of hematopoietic stem cells.
The machine learning approach allowed the team to create a clustering map of stem cells versus non-stem cells and track their behavior and differentiation over time. The approach assigned a score to determine whether a daughter cell is likely a stem cell or not, or whether the stem cells were dividing asymmetrically or symmetrically.
“It’s very exciting because we are not killing the cells. We are merely just taking images of the cell and then extracting those features. That can give us so much information about them.”
The team’s real-time approach to understanding the metabolic state of stem cells will provide further foundational knowledge that could aid drug discovery and cutting-edge stem cell treatments, as well as regenerative medicine treatments where human cells, tissues, and organs can be grown and replaced.
“There are other applications nowadays, such as cell therapy. People have been trying to make, for example, T cells, macrophages, and other types of cells that have their own specific utility in different kinds of disease contexts,” Shen said. “For stem cell people, this is an exciting technology because we allow them to look at a stem cell state in real-time and then track each cell over time, which is not currently possible.”
Published on June 18th, 2024
Last updated on June 18th, 2024