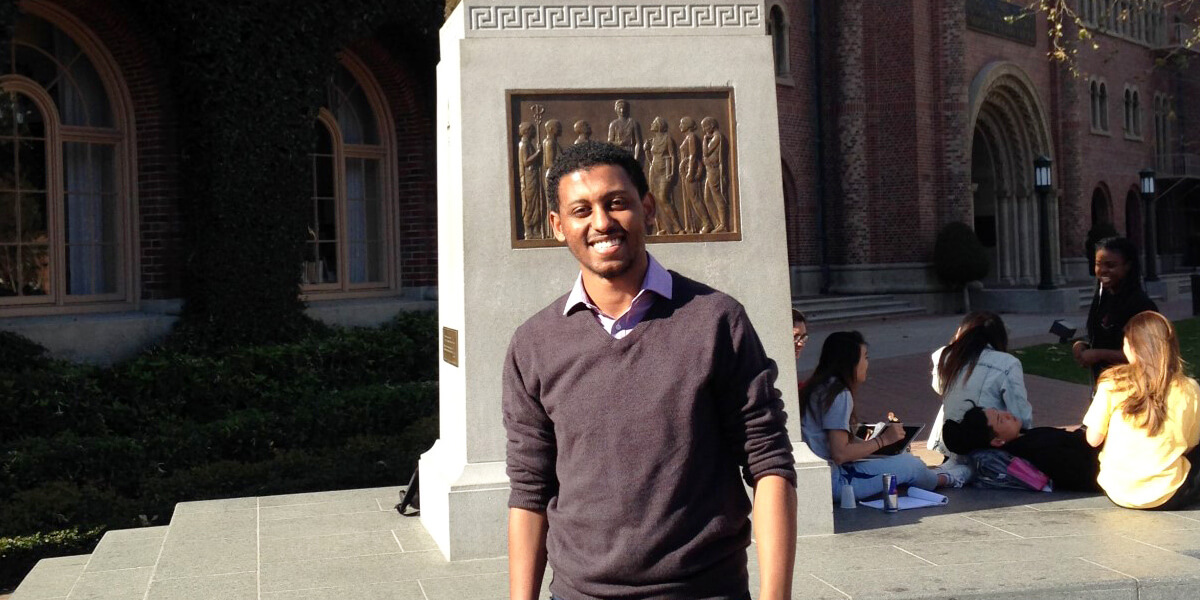
Basi Imana, who received runner-up in the Best Student Paper Award category at The Web Conference for his research on gender bias in social media job ads. Photo/Basi Imana.
You can’t apply for a job you’ve never seen. That, in a nutshell, is why Basileal (Basi) Imana is passionate about algorithmic fairness. The computer science Ph.D. student recently lead-authored a paper on gender bias in social media job ads, which found that Facebook algorithms used to target ads reproduced real-world gender disparities when showing job listings, even among equally qualified candidates
The research, co-authored by his supervisors Aleksandra Korolova, an assistant professor of computer science, and John Heidemann, a research professor at the Information Sciences Institute, has garnered interest in many publications, including MIT Technology Review, The Wall Street Journal, VentureBeat and The Verge.
Presented at The Web Conference 2021, Apr. 19-23, where Imana received runner-up in the Best Student Paper Award category, the study revisits a question tackled by Korolova and her colleagues in 2019: If advertisers don’t use any of Facebook’s demographic targeting options, which demographics will the ad delivery system target on its own?
In fields from software engineering to food delivery, the team ran sets of ads promoting real job openings at similar companies requiring similar skills—one for a company whose existing workforce was disproportionately male and one that was disproportionately female.
Facebook showed more men the ads for the disproportionately male companies and more women the ads for the disproportionately female companies, even though the job qualifications were the same. The paper concludes that Facebook could be violating federal anti-discrimination laws.
Imana, who is originally from Ethiopia and completed his undergraduate degree at Trinity College in Hartford, CT, specializes in algorithmic fairness. We spoke with Imana over Zoom about how automated decision-making can perpetuate inequality and what social media platforms could do to ensure fairness in algorithms.
When did you first encounter issues of fairness in algorithms?
My first exposure to the issues around algorithmic fairness was through reading the book Automating Inequality by Virginia Eubanks. The book uses case studies—one of which is about algorithms used to match homeless people to housing in LA—to explore how automated decision-making can disproportionately affect the poor. The book made a very compelling and eye-opening argument that made me interested in studying the societal and ethical implications of algorithms.
What, in your mind, is one of the most egregious instances of algorithmic bias or harm?
One that comes to mind is a study published in Science journal on an algorithm used by hospitals in the U.S. to determine which patients are high-risk and need extra monitoring or care. The algorithm was found less likely to flag eligible Black patients as high-risk because of how it uses previous healthcare spending as a proxy to calculate medical need. The researchers showed this results in disparity because people of color are more likely to have lower incomes and tend to pay for medical care less frequently.
In your latest research, you found evidence of gender bias in Facebook advertisement. Why is this an important finding?
It’s important because targeted advertising is ubiquitous—it affects millions of users, especially for high-stakes opportunities like employment, housing and credit. If human bias or historical bias is creeping through into these decision-making systems, they’re not going to help level the playing field. Instead, they’re going to perpetuate existing stereotypes. That’s why social media platforms need to re-examine their algorithms—ultimately, they’re shaping people’s access to opportunities.
“If human bias or historical bias is creeping through into these decision-making systems, they’re not going to help level the playing field.” Basi Imana
Can you give us an example of how bias in targeted advertising could impact someone’s job opportunities?
You can’t apply for a job you’ve never seen. Let’s say you’re a woman interested in being a medical doctor, but instead, you’re getting a lot of medical assistant ads because Facebook knows your gender and has learned that, historically, there are more female medical assistants. Basically, Facebook is deciding what’s interesting for you based on other people with similar behaviors.
That’s part of the problem: Facebook is saying, “we’re showing you relevant ads that are more interesting to you.” But by doing that, they’re defining what relevance means in more self-serving ways. The goal is to make it as relevant as possible from an advertising perspective. But should relevance always be the priority when the context is different? Should the same algorithm be used to decide who sees a job ad and a product ad?
What were some of the challenges you encountered while working on this study?
One of the challenges was coming up with a methodology to control for qualification: we were limited to the information made available for regular advertisers and we had to find data supporting the historical skew we wanted to test for. Also, we had to make sure we controlled for other confounding factors: we ran both ads at the same time, targeting the same audience, to control for factors such as competition for ads, or who tend to be online during the times the campaigns were run, since those are factors that can affect the outcomes as well.Â
Did anything surprise you about the results?
There are prior studies that audited Facebook and found evidence of bias, which the company said it would address. In that sense, it’s surprising that not much progress has been made. Much of the effort has been around the ad targeting tools advertisers can use. For example, you cannot target by age and gender for job or housing ads. But not much progress has been made in terms of what the algorithms are doing in deciding who sees a particular ad.
“As computer scientists, we need to think about the ethical consequences in our work if we want to live in a more just and equitable society.” Basi Imana.
How do we fix the problem? What is the first thing you would advise companies like Facebook to do to avoid algorithmic harm?Â
I think that’s an important question. It’s certainly not an inevitable problem: this is the outcome of Facebook optimizing a certain formula in their systems. And in theory, fairness metrics could be incorporated to decrease discrimination. But it’s not an easy issue because it affects business interests and relevance to users. So, there are a lot of different interests by different stakeholders involved that need to be considered. One main potential short-term solution could be disabling ad delivery optimization for job ads. There’s also a lot of work on fairness metrics, for instance the fraction of male and females that see a particular ad should not be too different. Basically, enforcing those constraints on whatever objectives they are optimizing for.
What is your hope for the future of algorithmic fairness? How do you hope to use your skills in the future to fight algorithmic harm? Â
I’m interested in privacy and fairness issues because it’s a high impact area and there are serious consequences of not solving this problem. As computer scientists, we need to think about the ethical consequences in our work if we want to live in a more just and equitable society.
I hope we, computer scientists, get to a place where considering the ethical implications of tools we build becomes an integral part of the software development cycle. By doing so, we can enjoy the benefits of advancements in algorithms and AI while using mechanisms at our disposal for minimizing social biases and harms.
Published on April 28th, 2021
Last updated on July 1st, 2021