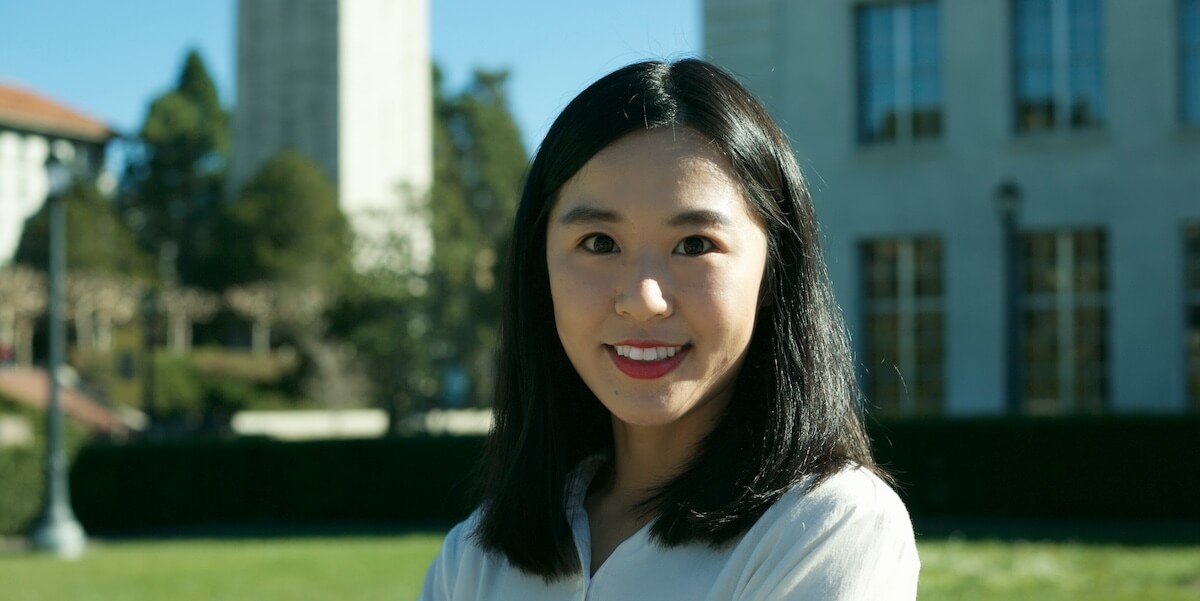
Renyuan Xu, WiSE Gabilan Assistant Professor in the Daniel J. Epstein Department of Industrial and Systems Engineering. Image/Renyuan Xu
Renyuan Xu has always loved challenging problems. As a child growing up in China, she developed a love of brainstorming questions, puzzles, and math contests. Now, as the newest WiSE Gabilan Assistant Professor in the Daniel J. Epstein Department of Industrial and Systems Engineering, Xu will bring her lifelong passion for problem-solving to lead research that harnesses probability and powerful machine learning tools to help us make better decisions in environments where there is uncertainty.
Xu’s current research focus is in the field known as stochastic control theory and game theory—which uses tools from probability to aid decision-making in complex scenarios. Her research covers areas such as finance, allocating resources for social welfare and COVID-19 public health policy.
“Probability theory is a tool to quantify uncertainty when we have multiple choices. Each choice will come with a possibility, so we can quantify the possibility of each outcome,” Xu said.
“Once we have some basic understanding about the practical decision-making problem we want to tackle, we can then formulate it as a mathematical problem and solve it with the most appropriate tools from stochastic analysis, optimization, and machine learning,” Xu said.
“Otherwise, we have so many different possibilities to try and it soon becomes intractable. Formulating a practical problem as a mathematical problem helps a lot in terms of making better decisions and this is one of the research strengths of our Epstein Department,” she said.
Xu comes to the USC Viterbi School of Engineering after a two-year role as a Hooke Research Fellow at Oxford University, in the Mathematical Institute, mentored by Professor Rama Cont.
“Oxford has one of the largest mathematical finance groups in the world,” Xu said. So I got the chance to work with incredibly talented researchers on the most challenging and innovative topics in mathematical finance. I was interested in how the models and analytical tools I previously developed could be applied to financial problems.”
In her recent work on understanding the borrowing and lending market from a theoretical game perspective, Xu and her co-authors identified several situations that may correspond to collusion behavior among larger financial institutions. Xu said the findings were useful in helping market regulators to decide when and how to regulate the lending market. The research also provides insights in market design, supporting the transition from the London Inter-Bank Offered Rate (LIBOR)—the benchmark interest rate at which major global banks lend to one another—to the Secured Overnight Financing Rate (SOFR), which is a median of rates that market participants pay to borrow cash on an overnight basis, using treasuries as collateral.
Xu’s work at Oxford also modeled the success of national versus local lockdown policies as a public health response to the COVID-19 pandemic, and how the potential benefits in terms of reduced fatalities compared to the economic impacts of lockdown policies.
“What we discovered was that local lockdown policies are more efficient, instead of having a national approach. We found there was heterogeneity across different locations because of the types of industries, lifestyles and age distribution in local areas,” Xu said.
“We also examined school closures with simulations to see if we could re-open schools back in August 2020, and we found this should not impact fatality rates too much,” she said.
Xu completed her undergraduate studies in mathematics at the University of Science and Technology of China. She first came to the U.S. for her Ph.D. at UC Berkeley, which she completed in the Department of Industrial Engineering and Operations Research under the supervision of Professor Xin Guo.
Most recently, Xu has been interested in how machine learning tools can be used so that the decision maker can learn from interacting with the unknown environment, in order to improve decisions. Her current research looks at applying these machine learning tools in a financial setting.
“Suppose we are looking at portfolio optimization and we want to find the best way to allocate our money, but most of the time we don’t fully understand the financial system because of the complex mechanisms and competition from other market participants,” Xu said. “Could we improve the portfolio allocation strategy by interacting with the financial system and getting feedback from the market?”
Xu has also been developing algorithms to apply in areas such as auction bidding for online advertisement placement. Each time that a user clicks on a site such as Amazon, the request is routed to an auction platform where advertisers who are interested in the user’s profile will bid for the advertising slot.
“But there are only a few milliseconds between our request and the appearance of the webpage, so the platform needs to figure out which type of advertisements they want to put on the webpage very efficiently,” Xu said.
Xu said she was looking forward to working with colleagues in the Epstein Department, especially given the department’s research strengths in areas such as resource allocation for social benefit, such as homelessness and healthcare policy.
“The department has a very strong group of people who are specialized in optimization and I would also like work on aspects from that perspective to integrate that into decision-making problems,” Xu said.
Published on October 25th, 2021
Last updated on October 25th, 2021