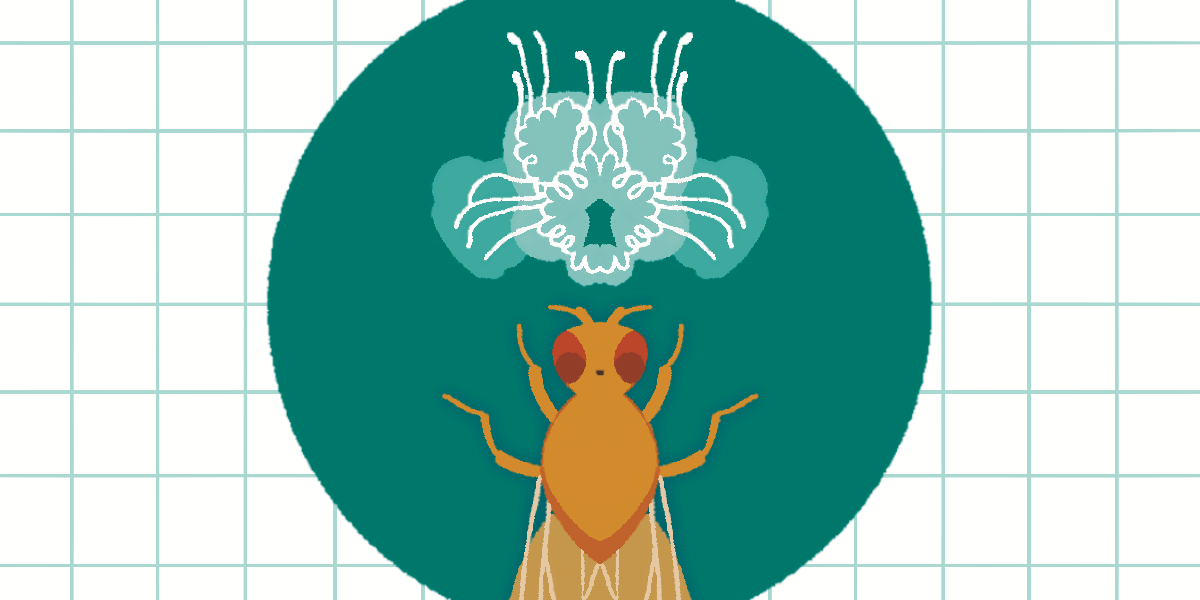
By applying their tool to, among other things, insect brains, the researchers were able to more deeply understand how our biological neural networks function (PHOTO CREDIT: Chris Kim)
Are you ready for a story about graphs?! Graphs are amazing things. Any complex network can be represented in a graph form; a social network, a financial network, a criminal network. In fact, with the right information and tools, a graph can see something even our best microscopes can’t. The graph in question for this story was developed when a simple question was asked: If you can only see the network as a whole, and not the individual nodes that make up the network, can you learn something about those individual nodes or a group of nodes and how they function over space and time?
New research from USC Viterbi has an answer for you. Yes, yes you can! The work, which appears in Nature Portfolio and is funded by the NSF and DARPA, is important for many reasons. It might allow us to better track and fight crime, monitor and remove misinformation on social media, or predict financial collapses. But as important as all those things are, the real groundbreaking application has to do with the most complex and misunderstood network in the known universe – the human brain.
This advance is important to solving one of the biggest roadblocks standing in the way of true AI: teaching machines how to generalize – to make analogies on the fly, in real-time, the same way people do.
“This is a tool to mine the biological complexity of the brain, discover its network generators (or the rules by which neuronal networks emerge for accomplishing a cognitive task), to mimic how it functions, and recreate that process for machine learning,” said Paul Bogdan, professor of electrical and computer engineering and lead researcher on the paper. In other words, the team’s work has gotten us one step closer to true artificial intelligence.
The team started by looking at the graph representation of the neuronal networks of an insect brain. By using their tool (a new algorithm) on a partially observed graph they were able to create a compressed representation of the insect neuronal networks and discriminate among brain regions where traditional network science metrics fail. Bogdan and his colleagues were able not only to understand how a bug distinguished between different things it encountered but also how it made classifications between say food, danger, or mates.
But that’s not all. The team’s tool improves a nascent area of research known as chromatin conformation analysis. This area of research looks at chromatin – the material that makes up an organism’s chromosomes. Chromatin contains DNA, RNA, and proteins that are constantly moving within a nucleus. Using their tool, the team can analyze and detect the 3D structure of chromatin and map it out in a graph. With this knowledge, we can better understand how new diseases emerge and better prepare for the future.
“You can think of this tool as something like a backdoor to understanding complex systems,” said PhD student Ruochen Yang, a researcher on the project. “We don’t have the technology to look at neurons that closely, but our algorithm and graphs allow us to understand them even without seeing them.”
This advance is important to solving one of the biggest roadblocks standing in the way of true AI: teaching machines how to generalize – to make analogies on the fly, in real-time, the same way people do. Our best machines still can’t really do this, meaning they still can’t truly think. But if we can better understand how we do it, that model could theoretically be recreated in a machine.
The team now plans to improve their analyses of these complex systems with even better tools that, quite honestly, push the boundaries of our imagination. First, they hope to apply a more dynamic version of their current model to networks to predict what they will do in the future. In other words, predicting the future. Second, they hope to apply the model on a deeper level to understand how individual neurons perform self-organization. If that process can be recreated in an artificial brain, it would allow an AI algorithm to rewrite itself when posed with a new task.
Bogdan is quick to point out the mathematical beauty behind his team’s work. “Having a mathematical strategy that can infer the network generator and so the rules by which a neuronal network emerges in a neural population can shed light on the cognitive performance and cognitive function even if only a small percentage of neurons are observed. This can circumvent or enhance our understanding where brain scan, microscope, or imaging technology provides only an incomplete picture of the large dynamic neuronal populations,” he said.
Published on December 3rd, 2021
Last updated on May 16th, 2024