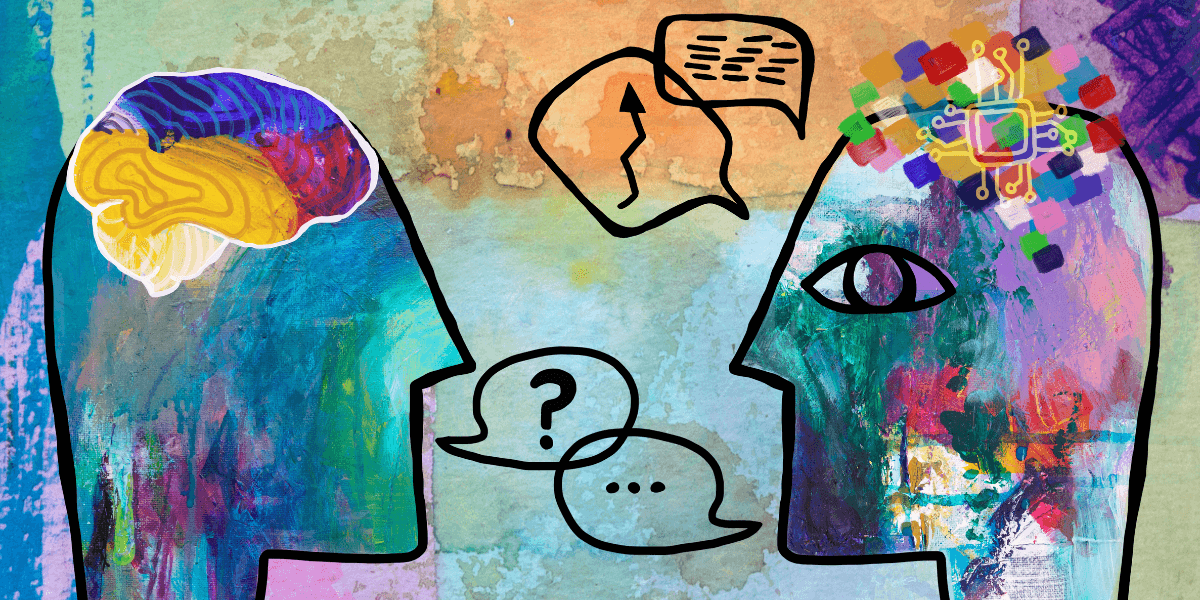
stellalevi/iStock
It’s common sense. Breakups hurt. Spilled water makes the floor slippery. Winter feels colder than summer. Understanding this type of knowledge has long been considered an innate human ability, honed through our everyday life experiences. But following rapid progress in transformer neural networks, large language models, such as BERT and GPT-3, are getting better at their own version of “commonsense reasoning.” Some even demonstrate human-like performance.
Does this mean that AI is ready to replace us in certain language-based tasks? Not yet, according to a new study that delves into evaluating how language models reason about common sense. Led by Ke Shen, a Ph.D. student at USC Viterbi and a Research Assistant with ISI, the paper, titled “The Generalization and Robustness of Transformer-Based Language Models on Commonsense Reasoning,” was recently presented at the Association for the Advancement of Artificial Intelligence’s (AAAI) Doctoral Consortium on February 21st, 2024.
“Transformer-based language models have recently become very popular,” said Shen. “We wanted to diagnose the performance of these models to see whether they can be reliable in real world applications.”
To measure how well AI can reason about common sense, researchers often use multiple-choice Q&A tests, covering a range of everyday topics, from social to physical to temporal knowledge. Language models have gotten very good at answering these questions accurately. This suggests that they have some ability to reason.
But some experts speculate that such high performance is superficial. The model may merely be memorizing and repeating information, rather than learning and reasoning about knowledge.
To assess this, Shen first tested the models’ ability to generalize specifically about commonsense reasoning tasks, or apply learned knowledge to new and unseen situations. The experiments aimed to determine if RoBERTa, a transformer model, maintained its performance on other commonsense reasoning benchmarks that were not part of its training data. For instance, a benchmark fine-tuned on physical knowledge was tested with social knowledge instead.
But Shen found significant decreases in RoBERTa’s performance when exposed to the unknown. This demonstrated that the models were not able to generalize well about common sense—an essential capability for AI to navigate the complex real world.
Next, Shen considered another concern with commonsense reasoning: overconfidence. Typically, a model assigns a confidence score to each of its predictions, which are taken as a measure of its certainty regarding the accuracy of the answer. But what if there are no right answers among the options? “In real life, sometimes you cannot give a confident prediction or answer,” Shen said.
Shen examined this in an experiment that modified the Q&A tests to be more confusing. Each question had its correct answer replaced with an incorrect one, meaning none were theoretically correct. In this scenario, models should return the same low confidence score for each potential answer. However, the study found that the models still indicated a clear preference for one answer—even though it was wrong.
This could cause problems in the real world. The ability for a model to flag the uncertainty of its answer could be important for something like a healthcare chatbot, says Shen. Imagine a patient provides the chatbot with a list of symptoms to gain a diagnosis, but the answer is unclear or complicated. A model that feels confident about an ambiguous answer is potentially harmful. “It’s better to hand these risky instances over to a human expert who can answer correctly,” Shen said.
To help guide this process, Shen has proposed a new, two-part framework that improves models’ ability to recognize when their judgements are likely to be incorrect or untrustworthy. This will determine how they should act. Right now, language models—such as GPT-3.5 Turbo, the underlying model of ChatGPT—have the capability to answer ‘I don’t know’ in risky instances, but they still choose to answer over 80% of the time, according to Shen.
The novel framework may help provide more nuance. In experiments, it led models to answer an extra 20.1% of “low-risk,” or fairly straightforward, commonsense reasoning questions. Perhaps more importantly, though, it also led them to abstain from 19.8% of “high-risk,” or complex and ambiguous, cases that they would have gotten wrong.
“We want to use this risk-adjusted framework to help the model identify: this is a risky instance, and I should not answer,” Shen said.
Published on February 20th, 2024
Last updated on February 23rd, 2024