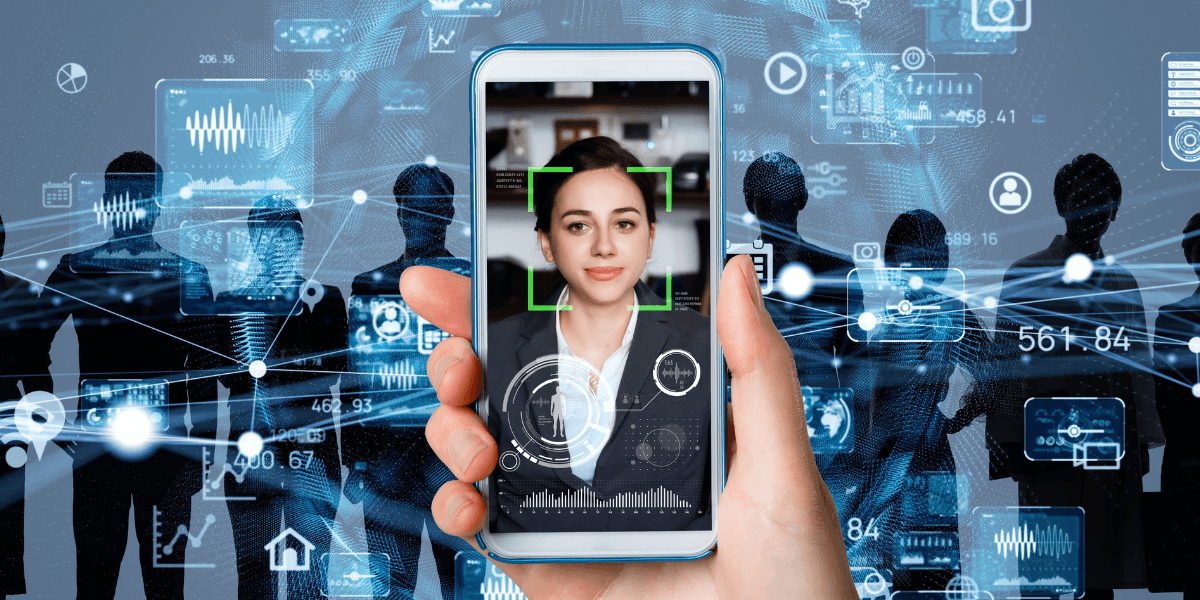
Photo Credit: Metamorworks/Getty Images
“Generative AI models are essentially methods that look at some data and try to create more of that data. Accurately measuring the performance of these models has become increasingly important due to the rapid growth of their application in downstream tasks,” said Mahyar Khayatkhoei, a computer scientist at USC’s Information Sciences Institute (ISI).
At the 40th International Conference on Machine Learning (ICML ‘23), held July 23rd to July 29th in Honolulu, HI, Khayatkhoei, who works with the VIMAL (Visual Intelligence and Multimedia Analytics Laboratory) research group at ISI, presented his latest paper on the performance of generative models.
Khayatkhoei said, “Performance is not usually something people look very closely at. They rely on benchmarks that exist and try to create better models, but it’s not always clear whether these models are really better. So, looking closely at what ‘better’ means, and whether the way you’re measuring that ‘betterness’ is accurate, is something that I think is very valuable.”
The paper is co-written by VIMAL founding director Wael AbdAlmageed, Research Associate Professor at USC Viterbi’s Ming Hsieh Department of Electrical and Computer Engineering and Research Director at ISI. AbdAlmageed said of the paper, “Generative AI is largely a poorly understood black box. In the middle of the hype about ChatGPT and large language models (LLMs), somebody had to slow down and try to study the behavior of these models in order to better characterize their performance.”
Generative Models, They’re Everywhere
A generative model was used to create an image of a black hole when scientists had parts of the image, and given those parts, the model was able to build out the rest. But generative models hit closer to home than black holes. Khayatkhoei said, “They are being used in many applications; many methods of image-based detection, for example, detecting cancerous tumors in a medical scan or human faces in photos, use some type of generative AI in their pipeline to improve accuracy; there are also direct use cases of generative AI in drug discovery, dynamics predictions, and Physics simulations.”
Khayatkhoei explained how: “We often don’t have access to as much data as we want, so we use generative models as a way to extend the number of observations that we train neural networks on.” Neural networks are the computing models used in AI that identify relationships in datasets.
An example: if you want an application to detect a cancerous tumor, the neural network must be trained on a very large dataset of tumors, and a generative model can create such a dataset. The quality of the generated dataset is described by fidelity and diversity.
How Good is Your Generative Model?
Khayatkhoei explains these attributes using the example of human face generation. “With generative models, we try to learn the distribution of data from a few observations. So a model might see a limited number of human faces and try to generate an infinite number of human faces. ‘Fidelity’ describes how realistic the images are. And then there is a question of how much ‘diversity’ the generation has; is the model generating the same face? Is it generating the faces of different shapes and colors and backgrounds, and so forth.”
A standard method of measuring the performance of a generative model is by quantifying fidelity and diversity using metrics called “precision” and “recall,” respectively.
Finding Flaws in Performance Measurements
In the paper, Khayatkhoei theoretically shows that there are flaws in precision and recall. “People use these measurements to create better models or to decide what model to use in their application. When these measurements are flawed, that means that all these decisions are potentially flawed as well,” said Khayatkhoei.
Khayatkhoei explained how he approached the challenge: “We created experiments to show that this issue exists, and we mathematically proved that it’s actually, under some assumptions, a very general problem. And then from the insights of the mathematical analysis, we created a modified version for calculating these metrics that alleviate the problem.”
Khayatkhoei presented his paper, Emergent Asymmetry of Precision and Recall for Measuring Fidelity and Diversity of Generative Models in High Dimensions, as a poster presentation at ICML ‘23.
He said, “I’m excited to talk to people about it and point out that these metrics might not actually be capturing what you think they’re capturing.”
ICML is one of the fastest growing AI conferences in the world. This year, the conference had a record-high 6538 submissions (a 16% increase from last year’s record-high of 5630), and an acceptance rate of 27.9%.
Published on August 2nd, 2023
Last updated on August 15th, 2023