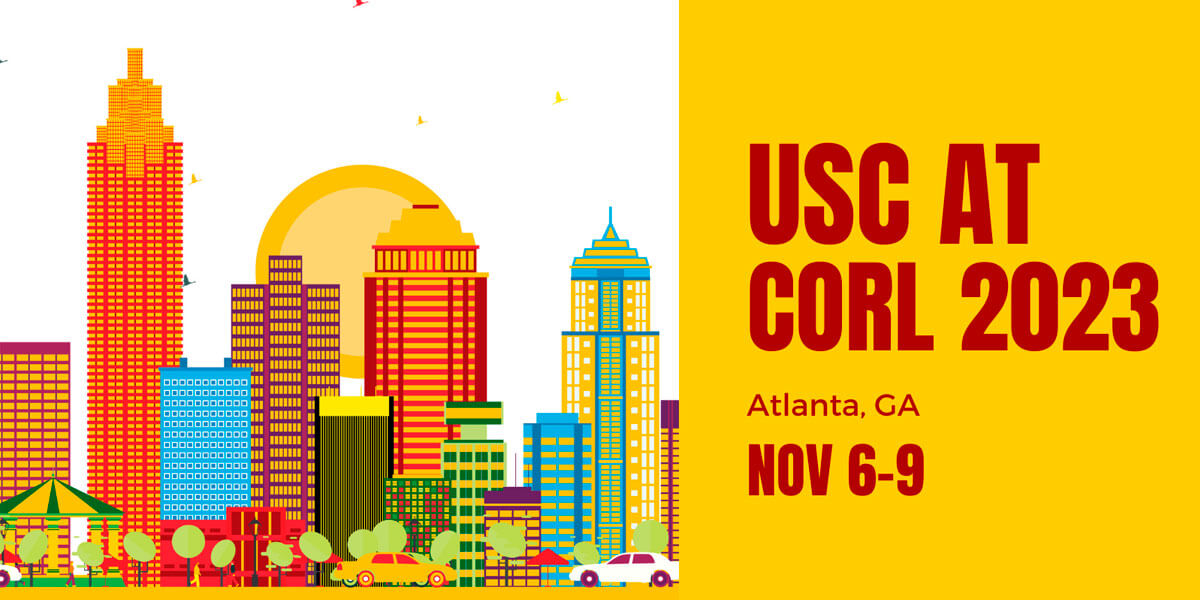
The Conference on Robotic Learning (CORL) is one of the largest and most influential robotics conferences. Photo/iStock.
This week, USC computer science students and faculty are presenting their latest work at the Conference on Robotic Learning (CORL), one of the largest and most influential robotics conferences. From finding and fixing faults in robots, to leveraging Large Language Models (LLMs) to guide robot learning, the research aims to advance the development of safer, more reliable robots, including household robots. Two exceptional papers have secured coveted spots in the conference’s oral track, with an acceptance rate of only 6.6%.
Putting robots through their pacesÂ
Imagine a household robot tasked with assisting in daily chores. Now, imagine this robot being deployed to millions of users. It would need to work well in different houses, perform different tasks, and interact with different users. “Our research aims to efficiently generate scenarios in which a given robot can fail. The robot designer can then look at these failures and fix the flaws before letting the robot interact with end-users,” said doctoral student Varun Bhatt, lead author of Surrogate Assisted Generation of Human-Robot Interaction Scenarios (oral track), advised by Stefanos Nikolaidis.
“Take, for instance, the behind-the-scenes footage of this humanoid robot that shows the robot falling multiple times during filming. Such mistakes can be dangerous if that robot is interacting with humans. We feel that our research is a step towards identifying the situations in which the robot will fail in human environments, making it easier for the engineers to isolate issues and fix them.”
The researchers present an efficient method of automatically generating challenging and diverse human-robot interaction scenarios. Video/Bhatt et al.Â
Learning new tasks in new settings
For autonomous robots to help humans perform useful tasks, they must be able to learn new tasks in new settings. “For example, a cooking robot trained at the factory should be able to quickly learn to cook relevant dishes in your apartment kitchen, even though it is a new setting with different types of dishes,” says Jesse Zhang, doctoral student and lead author of Bootstrap Your Own Skills: Learning to Solve New Tasks with Large Language Model Guidance (oral track), advised by Joseph Lim.
“Our project takes a step towards this goal by using Large Language Models, which have encoded world knowledge, to guide robots in learning to compose basic skills, like boiling water and baking vegetables, into useful, complex behaviors in new settings, like learning to make complex meals in your kitchen. [In the future,] humanoid robots, such as the Teslabot, will be able to navigate the world and will constantly encounter new settings and need to learn new tasks: our project could help them do so.”
Helping robots learn from other (different) robots
Let’s say you want to teach your robot how to fold a towel. But you have two hands, and your robot only has one. How will it learn to copy your actions? That’s the question tackled by Gautam Salhotra, a doctoral student advised by Gaurav Sukhatme, the lead author of a paper titled Learning Robot Manipulation from Cross-Morphology Demonstration.Â
“Previous research has methods to only partially solve the problem, but cannot extend to the more general cases. Our framework (MAIL) provides a way to do this,” said Salhotra, who also wrote a blog post on the topic. “We let the human teach the robot how to do the task (for instance, folding) with two hands. Then we use ‘trajectory optimization’ to convert the demonstrations into a robot doing it with one hand. Then, we take these converted demos, and run AI algorithms that do Learning from Demonstrations or LfD to create a more general policy that can work with variations, such as different sizes, thickness, and textures, for instance.”
The system successfully folds a flattened cloth into half, along an edge, using two end-effectors. Video/Salhotra et al.Â
Full list of accepted USC papers (USC authors’ names in bold)Â
Learning Robot Manipulation from Cross-Morphology Demonstration
Gautam Salhotra, I-Chun Arthur Liu, Gaurav S. Sukhatme
Cross-Dataset Sensor Alignment: Making Visual 3D Object Detector Generalizable
Liangtao Zheng, Yicheng Liu, Yue Wang, Hang Zhao
Surrogate Assisted Generation of Human-Robot Interaction Scenarios (Oral)
Varun Bhatt, Heramb Nemlekar, Matthew Christopher Fontaine, Bryon Tjanaka, Hejia Zhang, Ya-Chuan Hsu, Stefanos Nikolaidis
Bootstrap Your Own Skills: Learning to Solve New Tasks with Large Language Model Guidance(Oral)
Jesse Zhang, Jiahui Zhang, Karl Pertsch, Ziyi Liu, Xiang Ren, Minsuk Chang, Shao-Hua Sun, Joseph J Lim
Published on November 7th, 2023
Last updated on November 7th, 2023