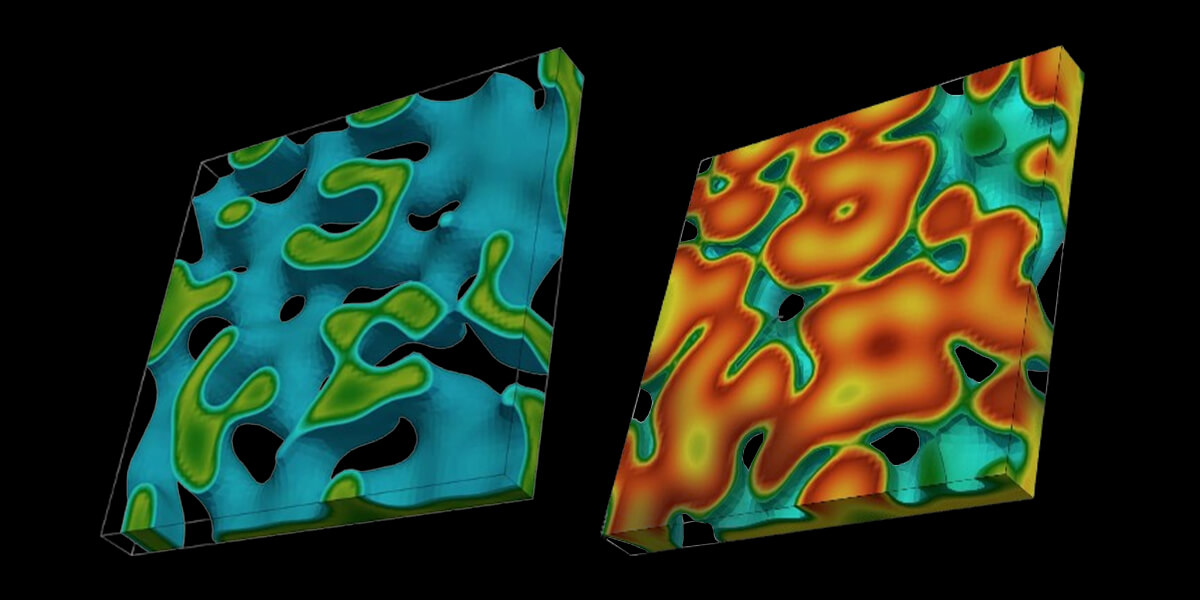
The formation of morphogenetic interfaces at anodes in solid state batteries. The colors signify concentrations of lithium and magnesium.
Have you ever witnessed a murmuration of starlings flying together at dusk? There’s something mesmeric about watching those patterns of grouping, splitting, swooping, rising… Could there be an inner logic hidden within those apparently chaotic formations?
The same is true of Professor Krishna Garikipati’s investigation of the patterns of motion and growth that describe cellular processes. For instance, during embryogenesis (the development of tissues that cohere to form an organism) or metastasis (the way that cancer cells migrate, multiply and evade treatment). This is how we live and die, and we see those dynamics playing out in the material world around us; from the way batteries charge, to the spread of pandemics.
Having previously led the Michigan Institute for Computational Discovery & Engineering at the University of Michigan, Garikipati recently joined the USC Department of Aerospace & Mechanical Engineering. His work is primarily computational, using techniques of applied mathematics – specifically, partial differential equations – to model the physics of living and non-living systems.
The curiosity equation
“Ever since my student days, it’s been a wonder to me that certain constructs in calculus have the capacity to accurately describe highly complex natural phenomena,” Garikipati recalls. “By regarding cells in terms of grouped populations, we can measure their reproduction and movement by using derivatives with respect to time and position. Essentially, partial differential equations represent how quantities change in time and space – and if you can develop and solve these equations, they describe a great deal of what’s happening.”
This ability to model the behavior of cells is particularly vital when it comes to combatting the migration of cancer cells – a process that is notoriously difficult to predict. Garikipati’s application of partial differential equations is also relevant to the complex physics of charge transport in batteries, a process that causes the battery to swell and shrink and ultimately determines its lifespan.
“Although I start from the standpoint of biology and the physics of life, my intellectual curiosity is constantly captured by how the mechanics of living organisms can be used to describe other material phenomena around us. This makes sense, if you think about it – a biological cell is essentially a little mechanical engine,” says Garikipati.
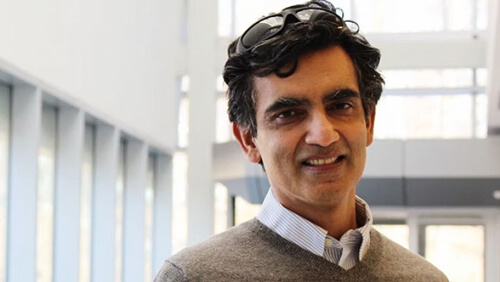
Professor Krishna Garikipati
“In the case of understanding energy transfer in batteries, it’s highly motivating to know that this work could feed into the development of more sustainable energy sources. Likewise, the same mathematical methods can used to understand weather patterns, the resilience of structural materials in the built environment, and even be applied to the unsolved mysteries of quantum mechanics.”
From matter to information
As a researcher who works in computation and prediction, the increasing sophistication of artificial intelligence and machine learning is tremendously exciting to Garikipati.
“The application of AI methods to understanding this physical basis of biology – and the wider applicability of those processes – is still in its infancy,” he explains. “We’ve barely scratched the surface of how AI algorithms could help us both to unravel that complexity through the formation and solving of equations, as well as enabling us to learn from the problems themselves. It turns out that we generate a lot of data as we produce the solutions, data that can then be used to solve new problems. In short, it’s an incredibly exciting time to be working in this area.”
The investigation of AI tools was a central focus of Garikipati’s leadership of the Michigan Institute for Computational Discovery & Engineering, and his work in the field was one of the primary reasons that he made the transition to USC Viterbi School of Engineering. The university’s recent launch of the Frontiers of Computing initiative and the founding of the USC School of Advanced Computing will be an unparalleled testbed for Garikipati to focus on research problems poised to break into new territory for AI and computation.
Systems thinking
The highly complex, interconnected problems that engage Garikipati’s mind are indicative of the way he likes to work. “Clearly, these types of topics are not for one person or group of specialists to do alone. Although the projects in my research group are computational and mathematical, we’re always collaborating with experimenters – whether cancer cell biologists, developmental biologists or materials scientists.
That’s one of the great things about USC: the different areas of expertise represented by the many centers and labs, and the culture of cross-disciplinary work advanced by Frontiers of Computing.”
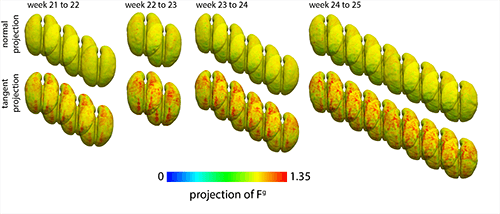
Inferred cell migration in the developing fetal human brain identifies the dominance of tangential crowding and predits the onset of the highly folded cortex.
“I believe that the recently launched School of Advanced Computing and the larger scope of the Frontiers of Computing have the potential to transform academia and to demonstrate how a university can have a significant impact on society,” he reflects. “At root, it’s a recognition that computation is taking place in every discipline, and that point of connection is worth cultivating with a $1 billion investment. A number of my colleagues here have put a great deal of thought into it, and the opportunity to be part of this moment, which comes but rarely in the life of a university, and to contribute to it made a compelling argument for me to move to USC.”
Naturally, Garikipati’s own interests were always going to extend beyond the specific focus of his own lab. The new influx of public access data collected during the Covid-19 pandemic (data banks that have further enhanced the accuracy of machine learning models) have prompted him to consider the relevance of his research to hazards engineering science.
“We’re surrounded by hazards: epidemics, wildfires, floods, earthquakes…” he observes. “There are models for each of these systems, and increasing interest in combining them to improve how we strategize and react. In addition, these physics-based models can be combined with agent-based models that predict the choice-behavior of individuals or groups. All this becomes very relevant to the current drive toward greater sustainability. How can we best deal with these challenges which arise from diverse sources and cross-react with a complexity beyond the scope of the human mind?”
Infinite application
“The back-and-forth between fundamentals and tangible application has been the defining feature of my career,” says Garikipati. “It’s true that working in academia affords us the luxury of hunkering down to focus on the abstract questions that intrigue us, and there’s a great joy in that. But for me, it’s always been clear that everything we do is embedded in society. We have the opportunity to recognize, react to, and even change what is happening in the wider world beyond the institution. I think we owe that to our students; to demonstrate the clear relevance of what they’re learning, and to take advantage of the waves of change that have the potential to shake up how we research and teach.”
A decade ago, that mindset prompted Garikipati to take advantage of the new network of “massive open online courses” (MOOCs) and to share his lectures in core subjects with a wider audience. In fact, he’s still something of a YouTube phenomenon. “Now, whenever I give a talk at the university or during a conference, grad students, postdoc or junior faculty will come up to tell me that they once studied those online lectures, or that they’re sharing them with their own groups and classes.”
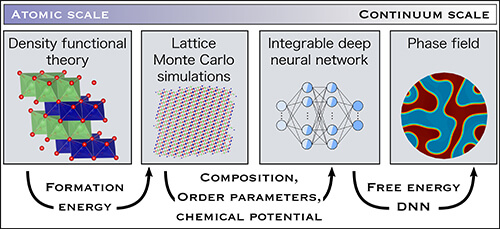
Scientific machine learning enables bridging from quantum to continuum scales to predict phase transitions in a lithium cobalt oxide battery particle.
Ultimately, Garikipati’s commitment to the wider application of his fundamental research is as much about his pedagogy as his active engagement in the development of new technologies. As he sees it, to teach is to provide a set of tools that equip students to find their place in the world – a process that starts by cultivating curiosity and opens the way to innovation. For Garikipati, that pedagogy finds its fullest expression in the School of Advanced Computing.
“Never before in academia, in science, engineering or any other field have we experienced such seismic change; change that’s affecting every facet of human society, from health, to entertainment, to transportation, to business, to finance. The projects in my lab are central to what’s taking place, both within Frontiers of Computing and society at large. I’m excited about what’s coming next.”
Published on March 19th, 2024
Last updated on April 24th, 2024